Exploring Databricks' Latest Features and Insights
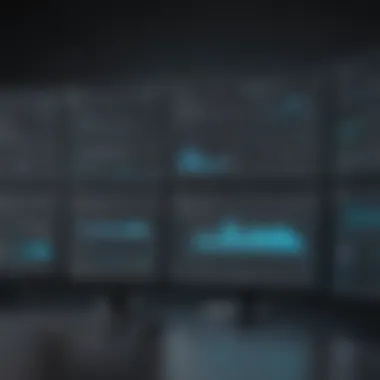
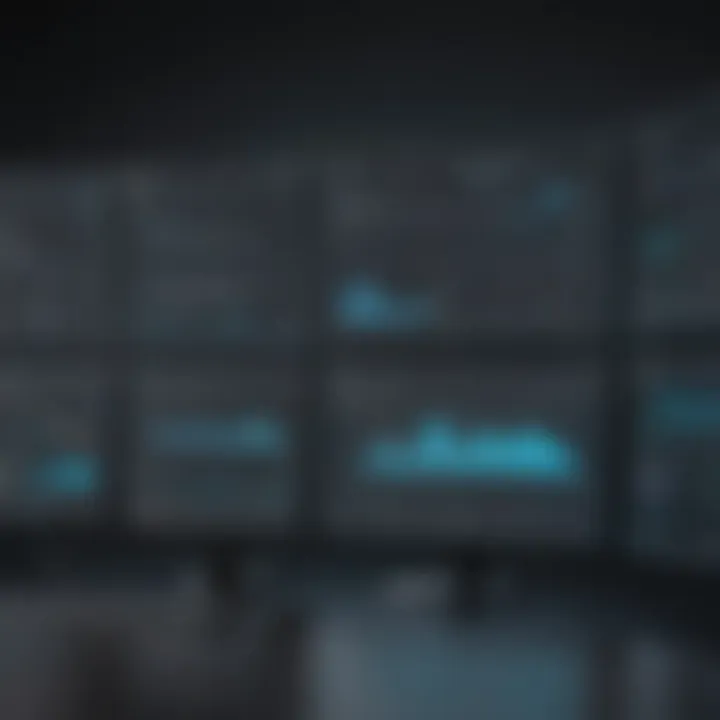
Intro
In the dynamically evolving landscape of data analytics, Databricks stands out as a formidable platform designed to enhance data processing and collaboration. Its recent features reflect a commitment to streamlining operations for data scientists and analysts alike. Understanding these updates is crucial for small to medium-sized businesses, entrepreneurs, and IT professionals. This article will explore the implications of these enhancements and provide insights tailored to the needs of decision-makers.
Software Overview
Purpose of the Software
Databricks was developed to simplify big data processing and analysis. It integrates capabilities of both data engineering and machine learning. This platform leverages Apache Spark, emphasizing speed and performance while allowing users to collaboratively work on shared analytics projects. By catering to diverse user requirements, Databricks is well-positioned to support organizations in harnessing data effectively.
Key Features
Recent updates to Databricks introduce several significant enhancements:
- Performance Improvements: The latest version optimizes processing speeds, allowing for quicker computations and reduced wait times for users.
- User Interface Enhancements: A more intuitive interface facilitates easier navigation, ensuring that both technical and non-technical users can utilize the platform efficiently.
- Integration Capabilities: Enhanced integration with various data sources and platforms allows for streamlined data ingestion and analysis processes. This includes support for commonly used data storage solutions.
These features aim to align Databricks with modern business needs, facilitating better decision-making.
"The effectiveness of data analytics tools hinges not just on their features, but also on their ability to integrate seamlessly within existing workflows."
Comparison with Competitors
Feature-by-Feature Analysis
Understanding how Databricks compares with its competitors can provide valuable insights into its market position. Platforms like Snowflake and Google BigQuery provide strong analytics capabilities as well. However, Databricks distinguishes itself through tight integration with machine learning workflows.
Some critical features to consider:
- Collaboration Tools: Databricks emphasizes real-time collaboration among teams, promoting knowledge sharing and faster problem-solving.
- Notebook Sharing: Users can share notebooks and visualizations easily, fostering an environment of continuous improvement.
- End-to-End Workflow Support: From data ingestion to model deployment, Databricks covers the entire analytics lifecycle, which is an advantage over many other platforms that focus only on one aspect.
Pricing Comparison
Pricing for Databricks can vary based on the features and scale of usage. Compared to Snowflake and Amazon Redshift, Databricks often provides a more flexible pricing model tailored to user needs. This can be beneficial for small and medium-sized businesses looking for an adaptable solution.
Intro to Databricks
Databricks serves as a vital resource in today's data-driven landscape. It integrates data engineering, data science, and analytics into a single platform. This approach is important for facilitating seamless workflows. Businesses that adopt such tools can harness complex data more effectively. When looking at the significance of Databricks, it is clear that it addresses more than just traditional data processing needs. It stands at the intersection of innovation and functionality. Navigating this complexity is essential for organizations that aim to maintain a competitive edge in their respective industries.
Overview of Databricks as a Unified Analytics Platform
Databricks is more than a data processing engine. It is designed to be a unified analytics platform that brings together disparate data sources. This integration simplifies the workflow for users, making it easier for teams to collaborate. The platform supports various data types, whether structured or unstructured. It is built on Apache Spark, which allows for big data processing capabilities. This foundation ensures that users can perform analytics in real-time, which is a crucial aspect for many businesses.
The collaborative features built into Databricks enable data scientists and engineers to work on shared projects. This fosters a more agile and responsive approach to data analytics. Organizations can analyze data insights quickly and adapt their strategies accordingly. Moreover, the user-friendly interface helps teams dive into their datasets without extensive training. This accessibility is beneficial for small to medium-sized businesses, where resources may be limited.
The Importance of Continuous Innovation in Data Analytics
Continuous innovation in data analytics cannot be overstated. The landscape is constantly changing due to advances in technology and shifts in market needs. Companies that do not keep up risk being left behind. Databricks recognizes this trend and continuously evolves to meet user demands.
Improvements in the platform enhance not only performance but also security and user adaptability. By implementing feedback from users, the developers ensure that Databricks remains relevant. This cycle of innovation leads to features that optimize resource use and improve efficiency.
"Continuous improvement is better than delayed perfection."
Performance Enhancements
Performance enhancements are crucial for any analytics platform, especially in today's fast-paced business environment. Organizations increasingly rely on data for decision-making. They need a platform that not only processes large volumes of data but does so swiftly and efficiently. Databricks addresses these needs with key improvements that optimize data processing.
Improvements in Processing Speed and Efficiency
Databricks has made significant strides in enhancing processing speed. This is achieved through a series of upgrades such as better resource allocation and advanced optimization techniques. For example, the latest engine version reduces latency and accelerates data retrieval times. This means that users can access insights faster, ultimately improving productivity.
Moreover, the platform employs strategies to optimize memory usage. Efficient memory management leads to less overhead, again speeding up processing times. This is particularly vital when handling complex queries or analytics on large datasets. By ensuring that system resources are used effectively, businesses can achieve better performance at a lower cost.
Another notable improvement is the integration of adaptive query execution. This feature allows Databricks to adjust query plans dynamically during execution, ensuring optimal performance even under varying workloads. With these enhancements, the processing of data becomes not only quicker but also more reliable.
Impact on Large-Scale Data Processing
The ability to process large datasets efficiently is a hallmark of Databricks. Large-scale data processing often poses challenges such as data bottlenecks and high computational costs. However, recent advancements in this platform significantly mitigate these issues.
First, scalability is a primary aspect. Databricks enables users to scale their operations seamlessly, accommodating the growing amounts of data without compromising speed. This is particularly beneficial for industries such as healthcare and finance, where data volumes are continually increasing.
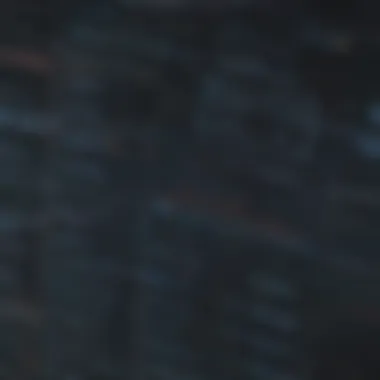
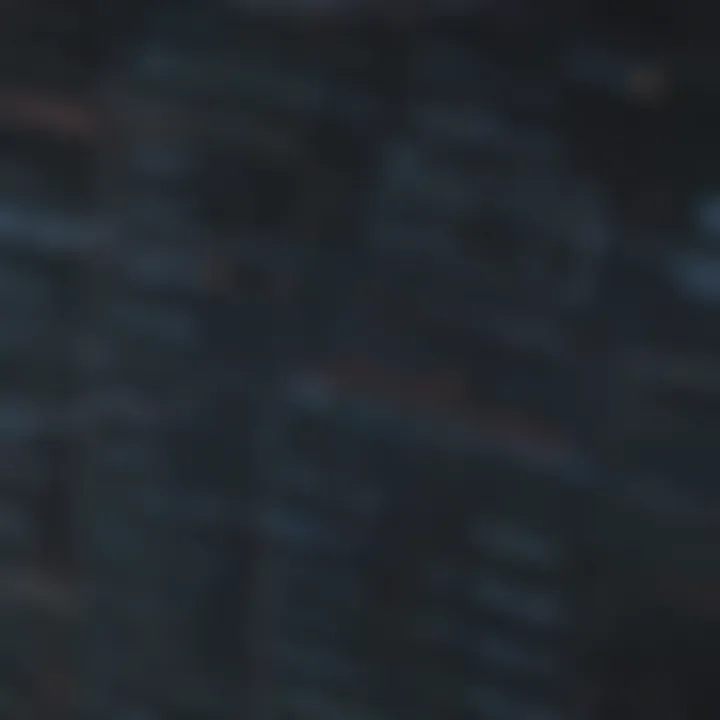
Second, improved parallel processing capabilities mean that tasks can be handled concurrently, reducing the total time required for analytics. This is key for businesses that depend on real-time data insights. Implementation of machine learning algorithms on substantial datasets can also yield faster results. Furthermore, efficient handling of big data leads to reduced operational costs. It lowers the resources needed for data storage and processing.
"In the world of data analytics, speed and efficiency are not just metrics. They are core to competitive advantage."
User Interface Enhancements
User interface enhancements play a pivotal role in the efficacy and usability of Databricks. An intuitive user interface is essential for improving user experience, expediting workflows, and enhancing productivity across various functionalities of the platform. These enhancements are particularly relevant for users who may not have deep technical expertise but need to engage with complex data analytics and data science tasks. A well-designed interface reduces the learning curve and aids in maximizing the utility of the features offered by Databricks.
Streamlined Navigation and Accessibility Features
Streamlined navigation is a cornerstone of user interface design in Databricks. It ensures a seamless flow from one feature to another, minimizing the time spent searching for tools or data sets. The focus on ease of access is particularly beneficial for small to medium-sized business professionals who often juggle multiple tasks and require quick access to insights. Visual hierarchy and logical grouping of features facilitate quick learning and familiarity with the platform.
Accessibility features are designed to cater to users with diverse needs. By adopting industry standards and guidelines, Databricks ensures compliance, thus fostering inclusivity. Key elements include keyboard shortcuts, screen reader compatibility, and adjustable settings for users with disabilities. This commitment to accessibility not only meets regulatory requirements but enhances the overall user experience.
Customizable Dashboards for User Personalization
Customizable dashboards represent a significant advancement in Databricks' user interface. Users can tailor their dashboards to suit unique workflow requirements, creating a personalized experience that resonates with individual preferences. This level of customization allows users to prioritize the information and visualizations that matter most to them.
For example, a marketing analyst may want to visualize sales performance data alongside social media metrics, while a data engineer might prioritize SQL query results. By enabling users to rearrange and select widgets, Databricks promotes efficiency and effectiveness in decision-making processes.
"The ability to customize dashboards transforms the way teams interact with data, making it relevant to specific roles and objectives."
Moreover, customizable dashboards support a dynamic work environment. As project goals evolve, so too can the dashboards. This flexibility is essential for organizations that need to adapt quickly in a competitive landscape, optimizing data usage effectively. In summary, the enhancements made in user interface design, particularly through streamlined navigation and customizable dashboards, offer significant benefits to users seeking to leverage Databricks for their data analytics needs.
Collaboration Features
Collaboration in the realm of data analytics is crucial for maximizing the potential of teams and leveraging the collective knowledge of an organization. Databricks has made significant strides in enhancing collaboration features, which are designed to promote teamwork and streamline project workflows. These features allow for fluid communication among data scientists, analysts, and business stakeholders. As organizations increasingly rely on data-driven strategies, effective collaboration can lead to faster insights and better decision-making.
One of the primary elements of these collaboration features is the ability to work on projects in real-time. This addresses a common challenge in data analytics where teams may be working in silos. With advancements in collaborative tools, input and feedback can be processed instantaneously. It fosters an environment where ideas can be shared freely and quickly, reducing delays in project timelines.
Real-Time Collaboration Tools
Real-time collaboration tools within Databricks enable users to edit notebooks and share findings instantly. These capabilities are particularly beneficial when working on complex data models or analyses. Teams no longer need to wait for asynchronous updates. Instead, multiple team members can engage on the same dataset simultaneously, bringing diverse perspectives to the analysis process. This real-time interaction is essential for ensuring that all team members have access to the latest information and can contribute their insights in a timely manner.
Moreover, features like comments, notifications, and version control enhance this experience further. Team members can leave feedback directly within the workspace, making it easier to track discussions and decisions. These enhancements streamline the workflow, eliminating the need for separate communication platforms and simplifying the overall user experience.
Implications for Cross-Departmental Projects
The implications of these collaboration enhancements extend beyond individual teams. Organizations often face challenges when multiple departments work on projects that require data insights. Databricks’ collaborative tools can bridge these gaps, ensuring that data from various departments can be integrated seamlessly. For instance, marketing teams can work alongside data scientists to develop more effective campaigns based on predictive analytics.
Cross-departmental collaboration can break down silos and foster innovative solutions.
Thus, businesses can leverage data from various sources, leading to more comprehensive insights. Improved collaboration also encourages a culture of knowledge sharing. When different departments collaborate work together, it promotes understanding and respect for one another's expertise.
Integration Capabilities
The integration capabilities of Databricks are crucial for sustaining a competitive edge in the domain of data analytics. Modern businesses thrive on diverse technology stacks. Therefore, having seamless integration across tools becomes a significant factor. This allows organizations to utilize their existing investments in technology while maximizing efficiency and productivity. Furthermore, effective integration can greatly enhance workflow processes and enable data-driven decision-making.
Seamless Compatibility with Other Tools
Databricks has taken significant strides to ensure seamless compatibility with a variety of essential tools. This includes popular data storage services like Amazon S3, Azure Data Lake, and Google Cloud Storage. The ability to connect with these platforms allows companies to readily access and analyze vast amounts of data without unnecessary delays or complications.
In addition, Databricks supports various business intelligence tools such as Tableau and Power BI. This integration means that users have the flexibility to visualize and interpret their data within the platforms they already use.
Here are some key benefits of this compatibility:
- Increased Flexibility: Users can collaborate using their preferred tools.
- Rapid Deployment: Integration reduces the time it takes to analyze data by connecting directly with existing infrastructures.
- Centralized Data Ecosystem: With better integration, companies can manage resources in a cohesive manner.
Leveraging APIs for Custom Solutions
APIs play a vital role in creating custom solutions tailored to specific business needs. Databricks offers robust APIs that empower developers to build unique applications that integrate directly with the Databricks environment. This empowers small to medium-sized businesses to innovate without the need for heavy investments in new software.
Utilizing these APIs can streamline workflows, automate repetitive tasks, and enable complex operations involving machine learning and data analysis. Benefits of leveraging APIs include:
- Customization: Businesses can tailor their applications according to specific processes and requirements.
- Scalability: As demands change, custom solutions can be adapted quickly.
- Enhanced Collaboration: APIs facilitate better communication between different systems, enhancing teamwork.
"Integrations and APIs unlock the door to tailored solutions that can evolve alongside your business needs."
Advanced Analytics Features
Advanced analytics has become a cornerstone for organizations seeking to harness the power of data. In the context of Databricks, the latest enhancements in this area underscore a significant shift towards automation and smarter decision-making. The combination of machine learning and predictive analytics provides businesses with tools that were previously the realm of complex statistical models. This chapter will dissect two primary facets of the advanced analytics features available in Databricks, illustrating their importance and potential impact on operations.
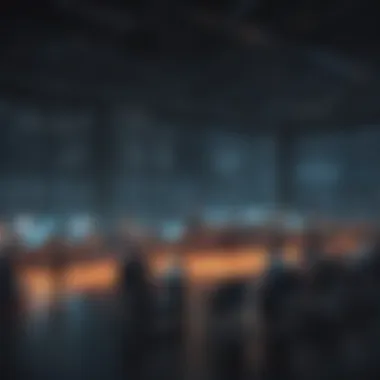
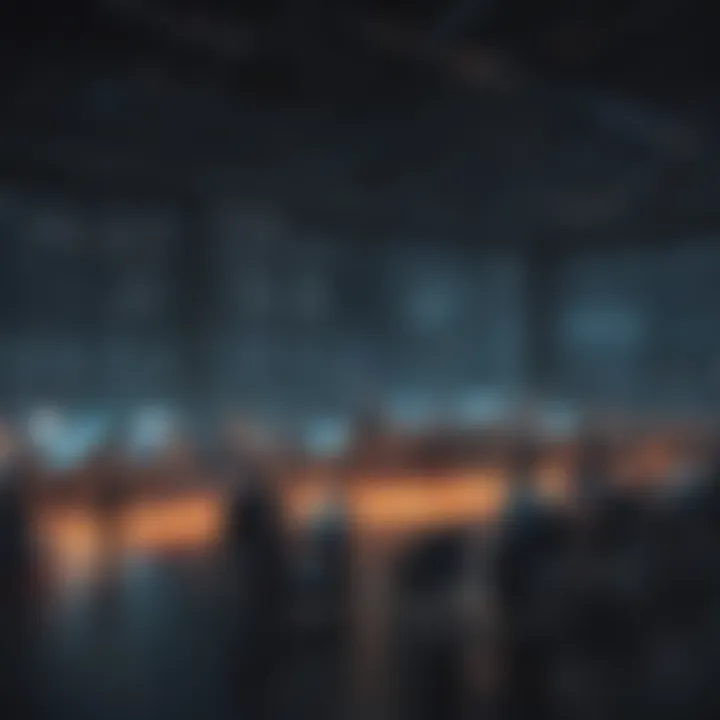
Prolusion of Machine Learning Capabilities
Databricks introduces robust machine learning capabilities that streamline the process of building, training, and deploying models. Traditional methods often require extensive resources and expertise. Databricks simplifies this through integrated tools that allow data scientists and analysts to collaborate effectively. The platform supports popular libraries such as Scikit-Learn, TensorFlow, and PyTorch, making it easier for professionals to apply machine learning techniques across various use cases.
This advancement means that small to medium-sized businesses can leverage sophisticated algorithms without needing deep technical knowledge. By providing features like automated ML and built-in notebooks, Databricks promotes efficiency in developing models. Users can focus on insights rather than getting bogged down by the complexities of coding. The result is not just faster development cycles, but also more informed business decisions based on accurate data predictions.
Predictive Analytics for Enhanced Decision-Making
Predictive analytics stands out as a transformative feature in Databricks. Organizations can analyze historical data to forecast future trends or behaviors. This capability is essential for decision-making processes across departments. For instance, marketing teams can anticipate customer needs, while finance departments can manage risks more effectively.
Key elements of
Predictive analytics in Databricks include:
- Data Integration: The ability to pull in different datasets allows for more comprehensive analyses.
- User-Friendly Dashboards: Visualization tools help present analytics intuitively, making insights accessible to all stakeholders.
- Real-Time Analytics: Companies can adjust strategies promptly based on current data rather than relying solely on historical patterns.
A noteworthy case study includes a retail company that successfully implemented predictive analytics using Databricks. By analyzing customer purchasing behavior, they optimized stock levels, significantly reducing overhead costs while increasing revenue through better inventory management.
"Predictive analytics provides actionable insights that inform strategy and foster data-driven decisions."
In summary, advanced analytics features in Databricks not only enhance the technical capabilities of teams but also empower businesses to make informed, timely decisions. As companies continue to collect massive amounts of data, these tools will become more crucial in maintaining a competitive edge.
Security Improvements
In the age of big data, ensuring the security of sensitive information is crucial. Security Improvements within Databricks address the challenges that organizations face in protecting data while still allowing for efficient analytics. These enhancements not only bolster the safety of stored data but also cater to the stringent requirements of data governance regulatory frameworks. Businesses acknowledge that safeguarding information can prevent data breaches, financial losses, and reputational harm. Adopting robust security measures can also enhance trust among clients and stakeholders.
Enhanced Data Protection Measures
The Enhanced Data Protection Measures in Databricks focus on multiple layers of security. These include strong encryption for data at rest and data in transit, ensuring that unauthorized access is minimized. With field-level encryption, users can manage the privacy of specific data elements, which is essential for sensitive information like personal identification data or financial records.
Additionally, Databricks implements fine-grained access control, allowing organizations to set precise permission levels for different users. This means that only authorized personnel can access certain datasets, reducing risks of internal leaks or misuse.
Organizations can also carry out audits and monitor logs to track access and changes to data. This creates a transparent trail of access events, which helps in identifying any suspicious activities quickly. Overall, these measures ensure that security is not just a check-box activity, but a foundational aspect of the data analytics environment.
Compliance with Regulatory Standards
Maintaining Compliance with Regulatory Standards is a priority for organizations that handle sensitive data. With the rise of regulations such as GDPR, CCPA, and HIPAA, businesses must ensure that their data practices meet these legal requirements. Databricks provides built-in features to assist organizations in achieving compliance.
The platform allows for easy data lineage tracking, meaning organizations can see where their data came from, how it has been used, and where it has been sent. This transparency is vital for compliance audits. Additionally, the integration of security features with regulatory frameworks means that organizations can automate many compliance tasks, reducing the manual workload and the potential for human error.
Furthermore, the frequent updates and security patches Databricks applies help stay ahead of potential vulnerabilities, aligning timely with compliance requirements.
"Proactive security measures in data analytics are not just best practices, they are essential for sustainable business operations."
In summary, the strength of Databricks' security improvements helps organizations protect their data effectively while ensuring compliance with essential regulations. These features are not only about safeguarding data but also about enabling business cryptography in a compliant manner, fostering a culture of safety and responsibility for data handling among users and stakeholders alike.
Cost Efficiency
Cost efficiency is a crucial aspect of assessing Databricks’s overall value proposition. In the landscape of data analytics, particularly for small to medium-sized businesses, budget constraints often dictate technology choices. Consequently, understanding the cost structure of Databricks and its potential benefits can drive informed decisions. Businesses can optimize their investment by evaluating what they get in return for their expenditure on this analytics platform.
Assessment of Pricing Models
When assessing Databricks's pricing models, it becomes clear that flexibility is a significant feature. The platform offers various plans, allowing organizations to choose what aligns best with their specific needs. Pricing can depend on several factors including the volume of data processed or the number of users. Some models may favor larger businesses capable of extensive data operations, while other options may cater to the distinct requirements of smaller enterprises. This layered approach enables businesses to minimize costs without sacrificing the quality of analytics services they require.
Key elements to consider in pricing models include:
- Usage-based pricing: Costs can scale according to actual usage, making it easier for businesses to control expenses.
- Subscription-based models: Offer predictable financial commitments over time but require careful evaluation of expected usage.
- Enterprise agreements: For larger organizations, these can provide additional discounts and dedicated support services.
Ultimately, businesses should carefully analyze their data needs and potential growth to select a pricing model suited to their trajectory. Making sure to account for future scaling can help avoid sudden financial strain as analytics demands evolve.
Long-Term Value Proposition for Businesses
The long-term value proposition of adopting Databricks extends far beyond mere cost evaluation. Investing in a comprehensive analytics solution can lead to significant returns through enhanced decision-making and operational efficiencies. By integrating advanced features like machine learning and predictive analytics, businesses can derive actionable insights from their data, leading to improved performance and profitability.
Considerations for long-term value include:
- Increased Efficiency: As processes become streamlined due to better technology, operational costs often decrease. This is crucial for maintaining competitiveness in the marketplace.
- Enhanced Decision-Making: The ability to analyze real-time data can lead to quicker, more informed decisions, which can significantly impact revenue and growth.
- Scalability: As businesses expand, the pricing models and features of Databricks can adapt to support growth without necessitating extensive retraining or overspending on new software.
A profound understanding of cost efficiency helps organizations not only to manage expenses but also to position themselves for growth accordingly. By evaluating pricing and weighing long-term benefits, companies can leverage Databricks to achieve strategic goals effectively.
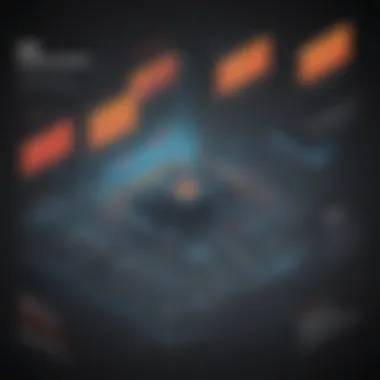
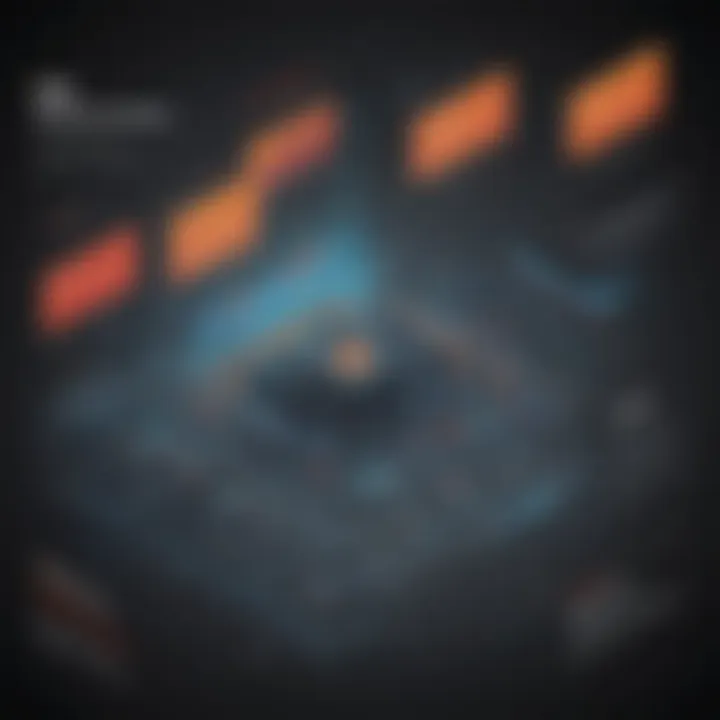
"Understanding cost efficiency is essential for leveraging technology in competitive markets. It’s not just about reducing costs, but maximizing value."
Organizations must thoughtfully consider their choices to take full advantage of the features that Databricks offers.
Use Cases and Practical Applications
Understanding the practical applications of Databricks is crucial for organizations that want to maximize their investment in this platform. The latest features introduced in Databricks greatly enhance the capabilities of users across various industries. By applying these features to real-world scenarios, businesses can improve their workflows, increase efficiency, and gain a competitive advantage.
The significance of this section cannot be overstated. It provides insight into how Databricks can be adapted to meet specific business needs. With the demand for data-driven decision-making on the rise, LEVERAGING these use cases effectively becomes vital for business growth. Deploying Databricks features will not only drive performance but also foster collaboration among teams.
Industries Benefiting from Recent Features
Multiple sectors are realizing the advantages of the recent updates in Databricks. Key industries include:
- Finance: Institutions are using enhanced machine learning capabilities to detect fraudulent activities more efficiently. The real-time processing of these datasets allows banks to act quickly and save resources.
- Healthcare: Data analytics in healthcare is critical. Hospitals and clinics leverage improved predictive analytics to better manage patient outcomes and optimize resource allocation.
- Retail: Retailers benefit from more sophisticated customer insights. The recent integrations allow for enhanced data processing of customer transactions, leading to more effective marketing campaigns.
- Manufacturing: Increased operational efficiency is achieved through predictive maintenance. Databricks supports complex analyses of machinery data to reduce downtime and improve production capabilities.
Each of these industries showcases how tailored applications of Databricks features can lead to substantial improvements in operational effectiveness.
Case Studies of Successful Implementations
To put the practical applications into perspective, let's examine some case studies that highlight successful implementations of Databricks's latest features.
- Financial Institution Case Study: A leading bank implemented Databricks to refine its risk assessment mechanisms. By leveraging the platform's machine learning capabilities, it created models that analyze vast amounts of transactional data. As a result, the bank reduced fraud detection time by 40% and significantly decreased operational costs.
- Healthcare Organization Case Study: A healthcare provider leveraged Databricks's advanced analytics features to improve service delivery. By deploying predictive analytics, they could foresee patient re-admissions and allocate staff efficiently. Their research noted a 20% decrease in unnecessary hospital stays, proving the effectiveness of analytics in enhancing patient care.
- Retail Chain Case Study: A major retail company adopted Databricks to analyze shopping patterns. The integration of data pipelines with customer data enriched their understanding of shopper behavior. This led to a tailored marketing strategy that increased customer retention by 15%.
These examples demonstrate the tangible benefits and efficiencies that Databricks can bring to diverse industries. Understanding such use cases encourages organizations to innovate and adopt data-centric strategies that are vital in today's business landscape.
Future Directions
Understanding the future directions of Databricks is integral for organizations and professionals aiming to remain competitive in a rapidly evolving data landscape. This section looks into anticipated developments and trends in data analytics that will shape how businesses operate and adapt their strategies moving forward.
Expected Developments in Databricks
Databricks is set to continue its trajectory of innovation. Key developments may include enhancements in machine learning capabilities, deeper integration with cloud services, and support for new data sources. Additionally, expect advancements in user interface design that enhance usability and reduce learning curves.
Another area to consider is the enhanced focus on automation. Businesses can expect features that allow for more efficient data pipeline construction and analytics workflows. The integration of artificial intelligence-driven tools will likely simplify complex tasks and increase productivity across various departments.
Benefits of these developments:
- Increased efficiency in data management
- Improved collaboration across teams
- Enhanced decision-making through advanced analytics
Emerging Trends in Data Analytics
The realm of data analytics is witnessing notable trends that resonate with the future directions of Databricks. One pivotal trend is the use of real-time data analytics. As organizations demand faster insights, technologies that process data in real-time are becoming critical. This aligns with Databricks' focus on streaming data and on-the-fly analytics, enabling businesses to respond immediately to changes.
Moreover, the emphasis on democratizing data access continues to grow. Business users, not just data scientists, are encouraged to engage with data analytics platforms. Features like natural language queries and user-friendly interfaces allow non-technical users to derive insights without heavy reliance on IT departments.
"The capacity to analyze vast data sets in real-time is no longer optional, but a necessity for modern businesses."
This shift has crucial implications for data governance and policy. Organizations will need to establish frameworks that ensure data is used responsibly while still allowing widespread access.
Other noted trends include:
- Increased focus on privacy and data protection
- Rising importance of data visualization tools
- Expansion of cloud-based data solutions
In summary, awareness of future developments in Databricks and the broader trends in data analytics equips businesses to leverage insights more effectively, thereby enhancing their strategic capabilities.
Closure
In this article, we have explored various aspects of the latest features offered by Databricks. Understanding these features is crucial for organizations aiming to improve their data analytics capabilities. The advancements in performance, user interface, and collaboration tools that Databricks provides can lead to significant operational enhancements. Companies leveraging these tools can extract more value from their data, ultimately driving better decision-making and fostering innovation within their teams.
Recap of Key Enhancements
Several key enhancements have been highlighted in this discussion:
- Performance Improvements: New functionalities have drastically increased processing speeds, allowing for faster data analysis.
- User Interface Enhancements: The updated UI focuses on ease of use, making it accessible for users with varying levels of technical expertise.
- Collaboration Features: Tools for real-time collaboration enable teams to work together seamlessly, which is especially important in today’s remote-centric work environment.
- Integration Capabilities: Databricks’ ability to integrate with a variety of platforms provides flexibility for businesses to adapt their analytics solutions to specific needs.
These enhancements position Databricks as a competitive player in the data analytics landscape, helping businesses not only to keep pace but thrive in this fast-changing domain.
Final Thoughts on Leveraging Databricks for Business Success
To capitalize on the benefits of the latest features in Databricks, businesses must adopt a strategic approach. This involves training staff to utilize the tool effectively and ensuring a thorough understanding of its capabilities. The investment in Databricks can provide an impressive return, especially for small to medium-sized enterprises looking to maximize their data insights.
Key considerations include:
- Training and Support: Ensure that team members are well-versed in using Databricks. This may involve workshops or online courses.
- Continuous Learning: Keep abreast of new features and updates that are launched regularly by Databricks to maintain a competitive edge.
- Performance Monitoring: Regularly assess how the platform is impacting operational efficiency and make adjustments as necessary.
By taking these steps, organizations can fully harness the power of Databricks, ensuring that they derive the maximum possible benefit from this robust analytics platform.