Comprehensive Insights into Software Image Recognition

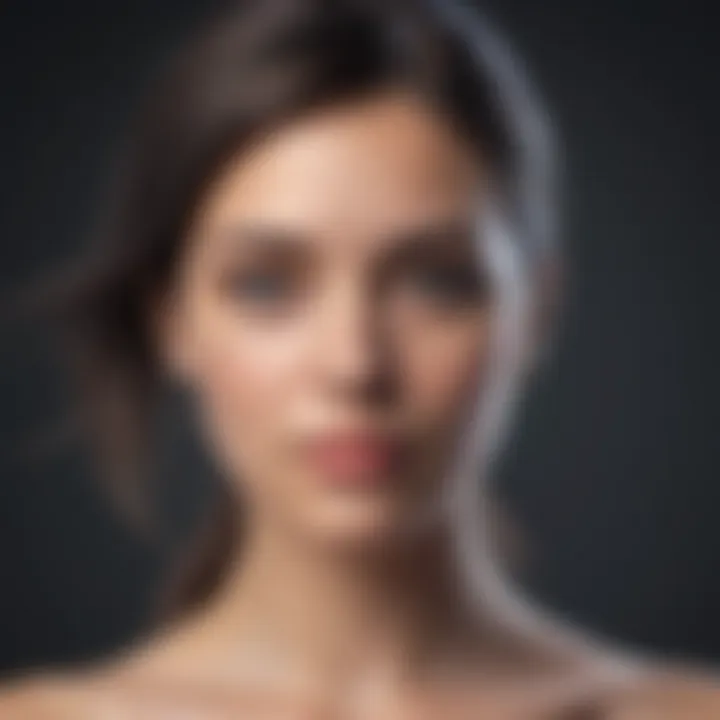
Intro
The field of software image recognition has seen remarkable advancements in recent years. With the rise of artificial intelligence and machine learning techniques, organizations across various industries are increasingly investing in this technology. Image recognition software enables systems to identify and process visual data efficiently. Understanding its capabilities is crucial for decision-makers, especially in small to medium-sized businesses and among entrepreneurs and IT professionals.
In this article, we will explore the multi-faceted world of software image recognition. The discussion will cover its core objectives, standout features, applications in diverse sectors, as well as the implications for businesses. We will also analyze market competition and emerging trends. Insights provided here will lend clarity to those looking to integrate image recognition into their operations or aiming to stay informed in a rapidly evolving landscape.
Software Overview
Purpose of the Software
The primary purpose of image recognition software is to transform visual information into actionable data. Organizations use this technology in several ways: automating routine tasks, enhancing customer experiences, and making data-driven decisions. For example, retailers might utilize image recognition to analyze customer preferences based on visual cues, like analyzing what products are most viewed or purchased based on images.
Key Features
Some key features of effective image recognition software include:
- Object Detection: The ability to pinpoint and classify various objects within an image.
- Facial Recognition: Identifying individuals through their facial features, often used in security or personalized marketing.
- Text Recognition: The capability to read and extract text from images, which streamlines data entry and analysis.
- Real-time Processing: Fast analysis of visual inputs to provide immediate feedback or results.
- Machine Learning Integration: Systems that adapt and improve accuracy over time, learning from new data inputs and refining their detection methods.
This robust functionality allows businesses to automate processes, enhance consumer insights, and ultimately drive sales and engagement.
Comparison with Competitors
Feature-by-Feature Analysis
The image recognition market is competitive, with several key players like Google Cloud Vision, Amazon Rekognition, and Microsoft Azure Computer Vision. Here’s a comparison of some features across these platforms:
- Google Cloud Vision: Offers robust image labeling, face detection, and landmarks recognition capabilities. The API is user-friendly and integrates well with other Google services.
- Amazon Rekognition: Known for its strong facial analysis features, including emotion detection and facial comparison. It also supports video analysis, making it versatile for varied applications.
- Microsoft Azure Computer Vision: Provides excellent optical character recognition (OCR) and image segmentation. It is particularly effective in processing forms and documents.
Pricing Comparison
Pricing models vary among competitors. Generally, it can be categorized as follows:
- Google Cloud Vision: Pricing based on usage, where costs are incurred per image processed.
- Amazon Rekognition: Offers a free tier with limited usage. Beyond that, charging is structured on a pay-as-you-go basis.
- Microsoft Azure Computer Vision: Similar tier-based pricing as well as scaling based on features used.
Comparing these offerings critically assists organizations in selecting the right software that aligns with their financial and technical requirements.
"The success of adopting image recognition depends not only on technology but also on understanding specific business needs and ethical implications."
Foreword to Software Image Recognition
Software image recognition refers to the ability of computer systems to identify and process images in a manner akin to human eyesight. This capability is central to many modern applications across various sectors, including healthcare, retail, and security. Understanding the importance of image recognition technology is essential, especially for small to medium-sized businesses, entrepreneurs, and IT professionals who aim to leverage these tools for competitive advantage.
The significance of software image recognition lies in its vast potential to enhance operational efficiencies, improve decision-making processes, and offer innovative solutions to longstanding challenges. For example, in healthcare, accurate image recognition can assist in early disease detection through analyzing medical images with unprecedented precision. In retail, it can personalize customer experiences by recognizing patterns in shopping behavior and preferences.
Considering software image recognition technology also entails acknowledging the challenges that come with its implementation. While the benefits are clear, organizations must navigate issues related to data quality and the integration of software with existing systems. Addressing these factors effectively can enable businesses to optimize their image recognition systems.
Fundamental Technologies Behind Image Recognition
Understanding the fundamental technologies behind image recognition is crucial for grasping how these systems operate. Image recognition refers to the ability of computer systems to identify and process images in a manner akin to human perception. This capacity is driven by various technological components that facilitate the analysis, classification, and interpretation of visual data. Each technology plays a distinct role, creating a synergy that enhances overall performance and application potential. By exploring these technologies, businesses can make informed decisions on implementing image recognition solutions.
Overview of Machine Learning Algorithms
Machine learning algorithms serve as the backbone of image recognition systems. These algorithms allow systems to learn from data rather than rely solely on pre-defined rules. With training data, machine learning models can discern patterns and relationships within images. The most commonly utilized algorithms include decision trees, support vector machines, and k-nearest neighbors.
The benefit of machine learning is its adaptability. As new data becomes available, the algorithms can recalibrate their understanding, leading to improved accuracy over time. However, it is essential to ensure that the data used is of high quality. Poorly curated data can lead to misleading results, emphasizing the importance of quality and quantity in training datasets.
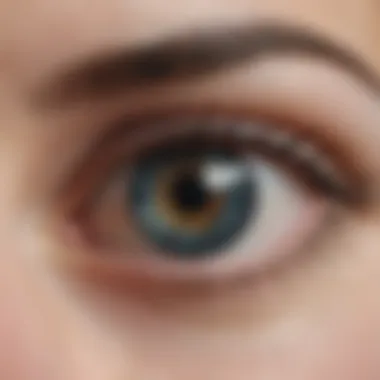
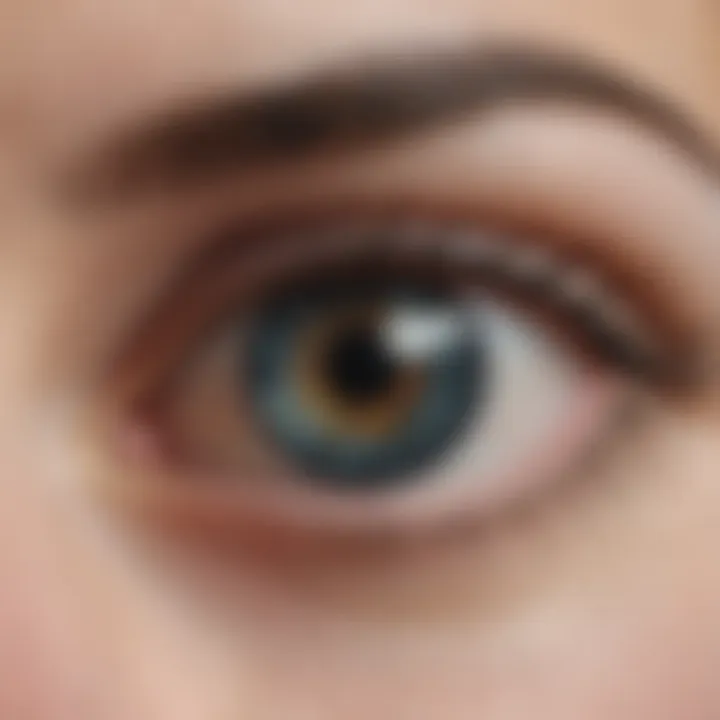
Deep Learning and Neural Networks Explained
Deep learning is a subset of machine learning that uses artificial neural networks with multiple layers. Unlike traditional machine learning models, deep learning excels in recognizing intricate patterns in large datasets, making it particularly useful for image recognition tasks.
Neural networks consist of interconnected nodes that mimic the human brain's functioning. In image recognition, convolutional neural networks (CNNs) are prevalent. These networks automatically identify features such as edges, shapes, and textures, thus allowing the system to grasp higher-level concepts. As deep learning models are exposed to more images, their predictive accuracy generally increases, paving the way for sophisticated applications.
The Role of Computer Vision Techniques
Computer vision techniques encompass methods that enable machines to interpret visual information. These techniques serve as crucial elements in the image recognition process. They facilitate the extraction and processing of features from images, enabling tasks like object detection and image segmentation.
For instance, techniques such as edge detection and feature matching allow computers to identify specific objects within a broader image. Continuously improving computer vision technologies contribute to the overall efficacy of image recognition systems. However, the integration of these techniques necessitates careful tuning and evaluation, as they can influence the depth and scope of insights derived from images.
"The synergy of machine learning, deep learning, and computer vision techniques underpins the evolution of image recognition capabilities. Together, they create systems that not only recognize but also understand visual content in valuable ways."
In summary, the convergence of these foundational technologies in image recognition holds significant implications for various sectors. By recognizing their importance, organizations can leverage these advancements to enhance operational efficiencies and innovate their service offerings.
Key Applications in Various Industries
The topic of key applications in various industries within the realm of software image recognition is crucial to understand. As organizations increasingly implement these technologies, they gain significant advantages across several sectors. Image recognition not only enhances operational efficiency but also catapults the customer experience into new realms. Moreover, it can lead to more accurate decision-making by providing actionable insights derived from visual data. Analyzing these applications helps businesses identify opportunities for advancement and potential pitfalls in deploying such technologies.
Healthcare: Revolutionizing Diagnostics
In the healthcare sector, software image recognition has proven invaluable for diagnostics. Medical imaging, including X-rays, MRIs, and CT scans, generates vast amounts of visual data. Advanced image recognition algorithms can assist radiologists by identifying anomalies that may not be immediately apparent to the human eye. This technology can reduce the time it takes for diagnoses, which can be vital in emergency scenarios. Furthermore, it plays a role in pathology by automating the analysis of tissue samples.
Here are some benefits of implementing image recognition in healthcare:
- Early Detection: Algorithms can highlight potential issues early in the diagnosis, such as signs of cancer.
- Increased Efficiency: Time saved in analyzing images can be redirected to patient care and other pressing tasks.
- Consistency: Machine learning models can maintain a consistent quality of analysis, minimizing human error.
However, challenges arise that must be addressed. Data quality, for instance, remains a significant concern. Inaccurate training data can skew algorithm predictions, necessitating rigorous data curation processes.
Retail: Enhancing Customer Experience
In retail, image recognition applications have transformed how businesses engage with shoppers. Through visual recognition, retailers can gain deep insights into customer behavior. Image recognition can automate inventory management by identifying products on shelves and suggesting restocking as necessary. Moreover, it enhances customer experience through features such as visual search.
For instance, customers can upload images of items they want, and the software can help find similar products available in-store or online. This capability leads to higher customer satisfaction and potentially increases sales.
Key advantages in retail include:
- Personalization: Tailoring experiences based on customer preferences gleaned from image recognition data.
- Operational Efficiency: Streamlining processes that would traditionally require manual oversight.
- Security: Reducing theft and ensuring better tracking through surveillance systems with image recognition integration.
Manufacturing: Streamlining Quality Control
Manufacturing environments benefit substantially from image recognition in quality control processes. Automated inspection systems can analyze products on assembly lines in real-time, ensuring they align with established specifications. Any defects can be quickly flagged, which minimizes waste and enhances productivity.
Image recognition can:
- Identify Defects: Point out minute flaws that the human eye might miss.
- Enhance Traceability: Linking images with historical production data can help track quality issues over time.
- Optimize Resource Use: By reducing faulty products, companies can significantly lower their material costs.
Despite these advantages, manufacturers must overcome integration challenges. Existing systems may not easily accommodate new image recognition technologies, requiring substantial investment in infrastructure.
Agriculture: Advancing Precision Farming
In agriculture, the advent of image recognition has introduced precision farming techniques that optimize yields and reduce resource waste. Drones equipped with image recognition capabilities can survey large fields. The analysis can identify crop health issues, water needs, and pest infestations.
Benefits of applying image recognition in agriculture include:
- Resource Management: More efficient use of water and fertilizers by accurately targeting application needs based on precise analysis.
- Yield Prediction: Enhanced abilities to estimate crop yields based on real-time visual data.
- Sustainability: Reduction of chemical use by applying treatments only where necessary.
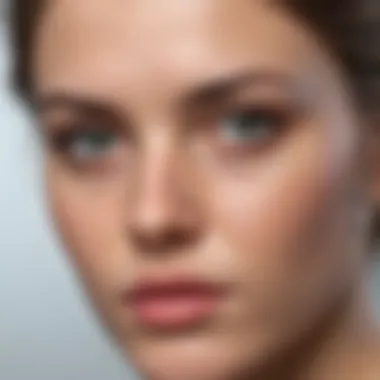
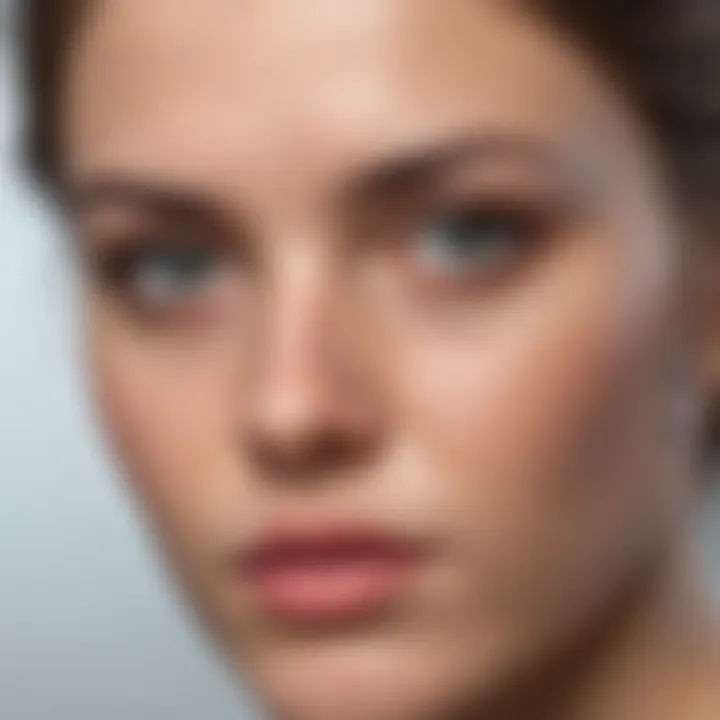
However, the implementation requires robust data strategies. Farmers must ensure they gather sufficient quality data to inform the algorithms accurately. This data can be complex and come from various sources, from satellite imagery to field sensors.
In summation, software image recognition finds transformative applications across various industries. From healthcare to agriculture, the impact is profound. As businesses recognize the potential, strategic implementation becomes increasingly vital.
Considerations for Implementing Image Recognition
Implementing image recognition technology entails several critical considerations. Understanding these factors can guide businesses in maximizing the benefits while minimizing potential drawbacks. As organizations explore the integration of image recognition software, the following key areas must be addressed: data quality and quantity, software selection, and integration with existing systems.
Data Quality and Quantity Issues
Data serves as the backbone for any image recognition system. High-quality data, consisting of diverse and representative images, is essential for training algorithms effectively.
- Quality of Data: Images must be clear and relevant to the tasks at hand. Poor-quality images can lead to inaccurate recognition outcomes.
- Volume of Data: A sufficient quantity of training data allows algorithms to learn patterns and improve their performance over time. Without adequate data, performance may suffer, leading to less reliable outputs.
Moreover, it is vital to consider the labeling of data. Accurate labeling informs the machine learning model about what to recognize in the images. Errors in labeling can propagate mistakes through the recognition process. Therefore, investing in a robust data management strategy is crucial for successful implementation.
Selecting the Right Software Solutions
Choosing the appropriate software solution is another significant consideration. The market is filled with various image recognition tools and platforms, each offering unique features and capabilities.
In this regard, businesses should focus on:
- Specific Use Cases: Consider what the primary purpose of implementing image recognition is. This could include security monitoring, quality control in manufacturing, or enhancing customer experiences in retail.
- Scalability: The selected software should support future growth and adapt to increased data or use case variations.
- User-Friendliness: Software that is intuitive and easy to use will facilitate better collaboration among team members.
Researchers and decision-makers are encouraged to review product demonstrations and seek user feedback before making a purchase decision to ensure alignment with organizational needs.
Integration Challenges with Existing Systems
Integrating image recognition systems with existing technology can present challenges. Compatibility issues may arise, complicating implementation and utilization. Notable integration aspects include:
- Infrastructure Considerations: Assessing whether current hardware can support new image recognition software effectively is critical. Upgrades may be necessary.
- Data Silos: Existing datasets need to be accessible and usable alongside new recognition systems. This requires proper data governance and possibly merging or restructuring data sources.
- Training Needs: Employees may require training to utilize the new system effectively. Ensuring that the workforce is prepared will lead to a smoother transition.
"Successful implementation of image recognition hinges on addressing data quality, selecting compatible solutions, and navigating integration challenges with existing infrastructure."
Evaluating Image Recognition Software
Evaluating image recognition software is a critical step for businesses aiming to leverage this technology effectively. The right software can significantly enhance operational efficiency and offer insightful data analysis. Companies must understand various factors, such as performance, user experience, and cost-effectiveness, to make informed decisions.
Performance Metrics and Benchmarking
When assessing image recognition software, performance metrics play a crucial role. Businesses should focus on accuracy rates like true positive, false positive, and overall classification performance.
- Precision and recall: These metrics help gauge how well the system identifies relevant data. Precision measures the accuracy of the positive results, while recall ensures that most of the relevant instances are captured. Evaluating these will help identify software that meets specific accuracy needs.
- Processing speed: It indicates how quickly the software performs tasks. Delay in processing can hamper business operations, making the software ineffective.
- Scalability: As a business grows, the demands on software increase. It is essential to choose a solution that scales efficiently without compromising performance.
"A software’s effectiveness is only as good as its performance metrics. Businesses must ensure these align with their operational goals."
User Experience and Interface Usability
User experience greatly affects how well staff can adopt and utilize image recognition software. A negative experience can lead to frustration and reduced productivity.
- Intuitive design: The software should be easy to navigate. Simple layouts help users understand and utilize features without extensive training.
- Customization options: Having the ability to tailor the interface to specific business needs is valuable. Businesses should evaluate whether the software allows personalization of features and modes.
- Training and support: Reliable customer support and comprehensive training resources can help users adapt quickly. This aspect reduces the initial learning curve and enhances overall efficiency.
Cost-effectiveness and Return on Investment
Cost plays a key role in selecting software. Analyzing cost-effectiveness is vital to ensure that a solution fits both budgetary constraints and business needs.
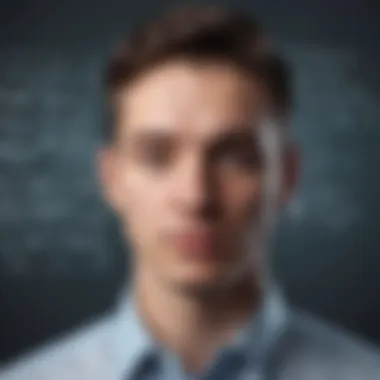
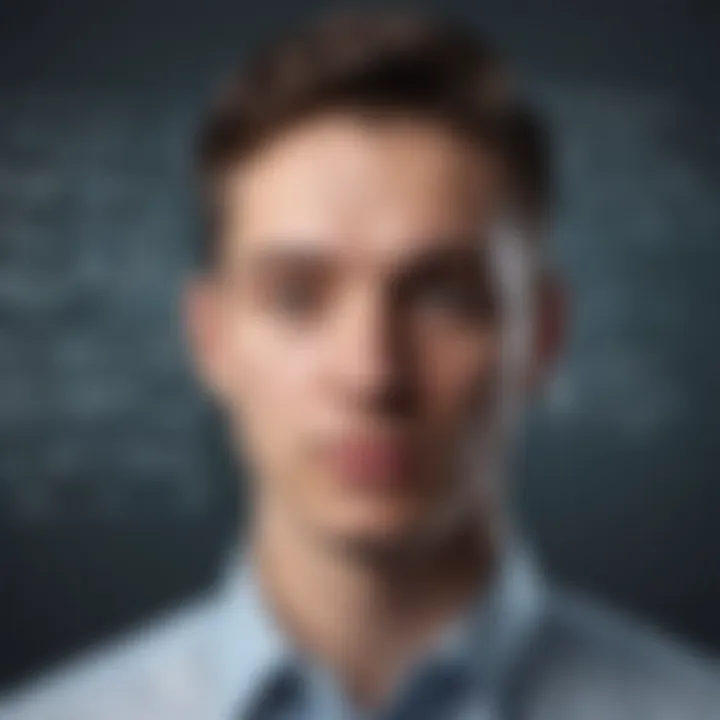
- Initial costs vs. long-term benefits: Businesses need to consider not just the upfront costs but also the potential long-term savings. Investing in high-quality software may be expensive initially, but the return on investment could prove beneficial over time.
- Licensing and subscription models: Different software solutions have varied cost structures. Understanding these helps in evaluating the overall financial impact, whether it be one-time purchases or ongoing fees.
- Impact analysis: Conducting thorough assessments of expected productivity improvements and cost savings resulting from implementing software allows businesses to justify their investment.
In summary, evaluating image recognition software involves a detailed analysis of performance metrics, user experience, and cost considerations. Small to medium-sized businesses must focus on these elements to select solutions that deliver real value.
The Future of Software Image Recognition
The realm of software image recognition is evolving rapidly. The future of this technology holds significant promise, especially for small to medium-sized businesses, entrepreneurs, and IT professionals. As organizations integrate image recognition into their operations, understanding the trends and developments will be crucial for maximizing benefits. This section addresses emerging technologies, edge computing advancements, and regulatory changes that will shape the landscape of image recognition in the years to come.
Emerging Trends in Artificial Intelligence
Artificial intelligence continues to push the boundaries of image recognition capabilities. One major trend is the improved accuracy of image identification through enhanced machine learning algorithms. Current models can recognize complex patterns effectively, contributing to diverse applications. Solutions leveraging transfer learning and automated machine learning significantly reduce training time and resource consumption. This acceleration makes it feasible for smaller companies to implement robust image recognition systems without extensive expertise in AI.
Moreover, developments in natural language processing are fostering richer interactions between users and software. For instance, integrating image recognition with conversational interfaces allows businesses to enhance customer service. This synergy improves problem resolution and enriches user experience, leading to customer satisfaction.
Potential Developments in Edge Computing
Edge computing represents a significant advancement for image recognition technology. By processing data at the source rather than relying on cloud services, organizations can achieve quicker responses and enhance privacy protection. This approach is particularly beneficial in industries like healthcare and manufacturing where real-time data analysis is critical.
The deployment of edge devices enables applications to function efficiently while ensuring high levels of accuracy. For example, retail stores can use edge computing to analyze customer behavior in real-time, allowing for immediate adjustments in service or inventory. The reduction in latency results in improved operational efficiency and a better overall experience for customers.
The Impact of Regulatory Changes
The landscape of software image recognition is not exempt from regulatory scrutiny. Privacy concerns regarding data collection continue to dominate discussions. Emerging regulations often aim to protect individual privacy rights and establish standards for ethical data use. Understanding these implications is vital for companies adopting these technologies.
Compliance with regulations such as the General Data Protection Regulation (GDPR) is essential. Businesses using image recognition must ensure that any data collected is processed lawfully. Transparent practices in software capabilities will not only facilitate compliance but will also build trust with users and stakeholders.
Understanding and adapting to emerging regulations is not merely a legal obligation; it represents a commitment to ethical standards in technology.
Ethical and Social Implications
The integration of software image recognition technology in various fields has brought forth a plethora of ethical and social implications that merit careful examination. The advancements in this domain offer remarkable functionalities, but they also pose significant challenges that can impact individuals and communities alike. Addressing these implications is crucial for businesses that are contemplating the utilization of image recognition systems. Understanding the ethical landscape not only promotes responsible deployment but also nurtures trust with users and stakeholders.
Data Privacy Concerns
Data privacy is one of the most pressing concerns when implementing software image recognition. This technology relies heavily on vast quantities of visual data, often collected from users without their explicit consent. For instance, facial recognition systems can analyze images from social media platforms, public spaces, or surveillance cameras. Such practices raise questions about whether individuals are aware that their images are being processed for commercial or security purposes.
It’s important to establish strong data protection policies. Organizations should be transparent about how they collect and use data. Implementing measures such as anonymization can help protect individual identities. Additionally, compliance with regulations like the General Data Protection Regulation (GDPR) in Europe is essential for avoiding legal repercussions and maintaining user trust.
"It is not enough to simply comply with the law. Companies must also strive to build confidence within the communities they serve."
Bias and Fairness in Algorithms
Bias in algorithms used for image recognition is a critical issue that can lead to unfair outcomes. For example, systems trained on datasets lacking diversity may fail to recognize individuals from certain demographics accurately. Such bias can exacerbate existing societal inequities, leading to discrimination. An illustration of this can be seen in facial recognition systems that often misidentify individuals with darker skin tones.
To combat this issue, stakeholders must ensure that training datasets are diverse and representative of the population. Regular auditing of algorithms can help maintain fairness, and companies should actively involve ethicists and social scientists in the development process. Addressing these biases is not only ethical but also enhances product effectiveness.
Accountability and Transparency in Usage
Accountability in the deployment of image recognition technology is crucial for ethical practices. When failures occur, whether due to false identifications or privacy breaches, it is essential to have mechanisms in place that allow for accountability. Users must feel secure knowing that companies are willing to take responsibility for their technologies.
Transparency likewise plays a fundamental role. Organizations should provide clear information about the technologies they employ, including how they operate and their implications for end-users. Publishing detailed documentation and engaging in dialogue with the public can foster trust and help demystify the technology.
Ultimately, companies should embed ethical considerations into their core business strategies. This approach not only mitigates risks associated with misuse but also promotes a culture of ethical awareness that benefits both the organization and society.
Ending
In this article, we explored software image recognition, shedding light on its applications and implications for businesses. This technology has become increasingly vital across sectors, from healthcare to agriculture. Understanding its core elements is essential for decision-makers in small to medium-sized businesses and IT professionals.
The importance of this topic lies in various key considerations:
- Technology Adoption: As industries evolve, the integration of software image recognition can optimize operations. Businesses must consider how these systems can add value to their processes.
- Strategic Impact: Understanding the strategic significance of image recognition can enhance competitiveness. Companies that leverage this technology effectively can differentiate themselves in crowded markets.
- Ethical Considerations: The ethical implications surrounding data privacy, bias, and algorithmic fairness demand attention. Businesses must navigate these issues to build trust with their customers and stakeholders.
- Future Outlook: Staying informed of emerging trends in artificial intelligence and edge computing will prepare business leaders for future developments. This awareness is crucial for ongoing adaptation and success in the long term.
Overall, the insights shared in this article provide a comprehensive understanding of software image recognition. This knowledge empowers decision-makers to make informed choices, enhancing productivity and innovation while considering ethical implications. In a rapidly changing technological landscape, a solid foundation in software image recognition is not just beneficial; it is imperative.