Harnessing SurveyMonkey and SPSS for Data Insights
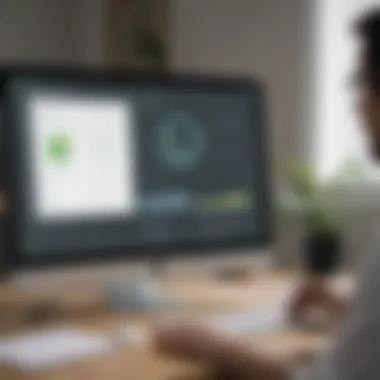
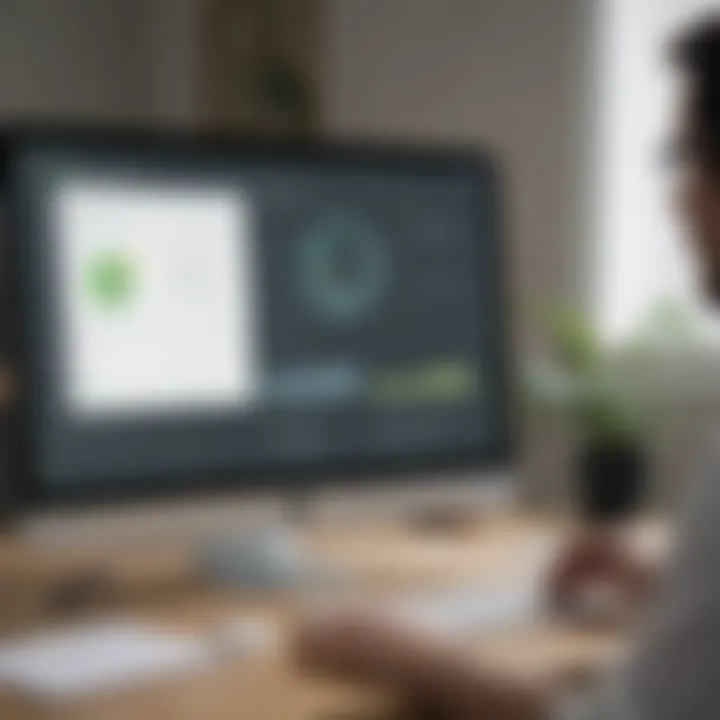
Intro
In today’s data-driven world, the need for effective tools for data collection and analysis has never been greater. SurveyMonkey and SPSS have emerged as significant players in this landscape. SurveyMonkey streamlines the process of gathering data, while SPSS excels at analyzing and interpreting those data sets. This article delves into how these two platforms, when combined, can enhance the capabilities of small and medium-sized businesses, entrepreneurs, and IT professionals seeking to derive insights from survey data.
Software Overview
Purpose of the Software
SurveyMonkey is primarily designed for survey creation and distribution. It provides an intuitive interface for users to design questionnaires that can be deployed across various channels. The flexibility of this software allows for customizing surveys to meet specific research needs. On the other hand, SPSS (Statistical Package for the Social Sciences) offers a comprehensive environment for statistical analysis. Its robust features enable users to perform various statistical computations, thus bridging the gap between raw data collected and actionable insights.
Key Features
SurveyMonkey boasts several key features:
- User-Friendly Interface: Allows users to create surveys without prior experience.
- Templates & Customization: A variety of templates are available, along with adjustable question types.
- Integration Options: Integrates easily with other platforms for streamlined data collection.
- Real-Time Analysis: Offers basic real-time data analysis to give immediate insights as responses come in.
SPSS offers powerful features as follows:
- Advanced Statistical Techniques: Supports complex analyses, including regression, ANOVA, and clustering.
- Data Visualization Tools: Facilitates effective presentation of results through charts and graphs.
- Output Management: Allows exporting of results in various formats for easier reporting.
- Extensive Documentation and Support: Available resources assist in learning and troubleshooting.
"Combining the ease of SurveyMonkey with the analytical depth of SPSS presents a unique advantage in data-driven decision-making."
Integration of SurveyMonkey and SPSS
The connection between SurveyMonkey and SPSS can significantly improve data utilization. Users can collect data easily through SurveyMonkey and later import it into SPSS for in-depth analysis.
This process usually involves exporting your SurveyMonkey results as a CSV file and using SPSS to perform more powerful statistical evaluation. Understanding this workflow allows organizations to efficiently leverage their survey data.
Comparison with Competitors
Feature-by-Feature Analysis
While SurveyMonkey and SPSS hold unique positions in the market, their features stand out when compared to competitors:
- Google Forms vs. SurveyMonkey: Both are user-friendly, but SurveyMonkey offers more advanced analytical capabilities.
- Tableau vs. SPSS: Tableau excels in data visualization, while SPSS leads in statistical analysis.
Pricing Comparison
SurveyMonkey offers multiple pricing tiers, which include basic free options, as well as premium plans that unlock more features for business use. SPSS, while generally more costly, justifies that expense through robust analytical power. It typically requires a subscription or license to access its comprehensive suite.
Preamble to SurveyMonkey and SPSS
SurveyMonkey and SPSS stand out as critical tools in the landscape of data analysis and research methodologies. This article delves into the synergy between these platforms, demonstrating how their integration can amplify data collection and analytical processes. SurveyMonkey offers a robust online survey framework, while SPSS provides powerful analytical capabilities for interpreting the collected data. Understanding both platforms adds value not only to researchers but also to businesses that aim to enhance their decision-making through data-driven insights.
Overview of SurveyMonkey
SurveyMonkey serves as a widely used platform for conducting online surveys. It enables organizations to design, distribute, and analyze surveys with ease. Users find its interface intuitive, which simplifies the process of gathering feedback or data from diverse audiences. The platform supports multiple question types, including multiple choice, open-ended, and rating scales, allowing for flexible survey design.
Among its features, SurveyMonkey offers tools for customization and branding. Users can adjust the appearance of surveys to align with their organizational identity, which can improve response rates. Additionally, SurveyMonkey provides real-time analytics, allowing users to view results as they come in. This capability helps in making timely decisions based on initial feedback.
Another noteworthy advantage of SurveyMonkey is its accessibility. The platform can be used across various devices, facilitating participation from a broader demographic. With options for anonymity and data protection, users are often more willing to share honest feedback, enhancing the quality of the collected data. Overall, SurveyMonkey serves as a foundational tool for effective data collection practices across industries.
Overview of SPSS
SPSS, known as Statistical Package for the Social Sciences, is a powerful tool for data analysis. It is widely used in both academic and professional settings for its vast array of statistical capabilities. With SPSS, users can perform complex analyses, ranging from simple descriptive statistics to advanced inferential tests. This flexibility makes it an essential resource for anyone needing to draw meaningful conclusions from their data.
The software supports various data formats, allowing users to import data from numerous sources, including Excel spreadsheets and databases. This feature is particularly useful when data is collected through platforms like SurveyMonkey. Once data is imported, SPSS offers a variety of analysis techniques such as regression analysis, factor analysis, and ANOVA, which can uncover underlying patterns and correlations.
Moreover, SPSS provides detailed output in the form of tables and graphs. These visual representations assist users in better understanding the implications of their data, offering clear insights for decision-making. Its extensive documentation and active user community further enhance the learning experience for new users. By combining SPSS's analytical capabilities with SurveyMonkey's data collection, researchers and businesses can achieve comprehensive insights that drive effective strategies.
The Importance of Data Collection in Research
Data collection serves as the backbone of research, playing a crucial role in shaping outcomes. The insights gleaned from data enable stakeholders to make informed decisions. This article highlights the significance of this topic, especially in the context of using SurveyMonkey and SPSS. Such tools enhance how data is gathered and analyzed, ultimately enriching the research process.
One of the primary reasons data collection is vital is its ability to provide empirical evidence. Decisions based on solid data are more likely to yield positive results rather than choices made purely on instinct or opinion. Moreover, robust data collection allows researchers to identify patterns and correlations. This analytical perspective is essential in numerous fields, including business improvement, marketing strategies, and social sciences.
Understanding Data Collection Techniques
Data collection techniques vary considerably. Each technique has its strengths and weaknesses, making it imperative for researchers to understand which methods suit their specific objectives. Surveys, interviews, observations, and experiments are among the most common approaches.
Surveys are particularly notable for their ability to reach large populations quickly. They often use questions that can be quantified, providing statistical support for conclusions. Interviews, on the other hand, offer deeper insights through direct dialogue but may involve complexity in analysis due to qualitative data. Observational methods can yield information about behaviors in natural settings, while experiments allow for controlled tests of hypotheses.
"The choice of technique directly affects the quality of data. Selecting an inappropriate method can lead to misleading results."
Employing the right data collection technique is a critical step that should not be overlooked.
Benefits of Using Online Surveys
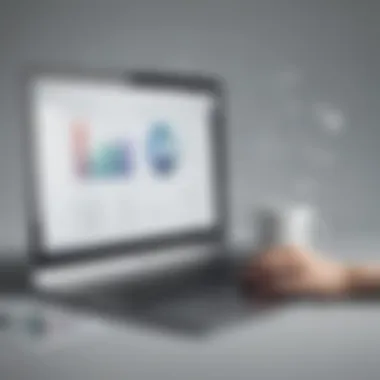
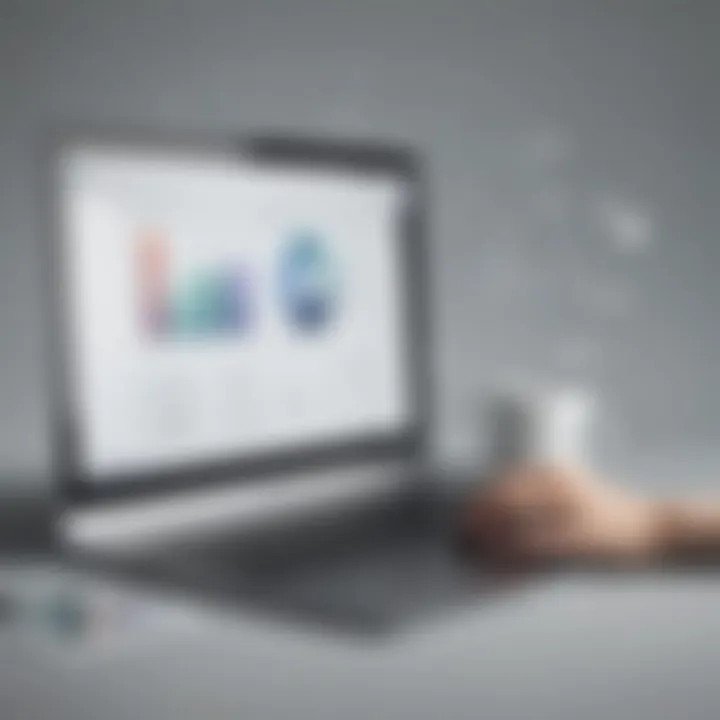
Online surveys, like those created with SurveyMonkey, present numerous advantages that enhance the efficiency and effectiveness of data collection.
- Cost-Effective: Online surveys reduce costs associated with printing and distribution. They also minimize the need for physical space to conduct surveys, making them suitable for tight budgets.
- Reach: These tools have the potential to span geographical boundaries, gathering responses from participants all over the world.
- Quick Data Collection: Obtaining results becomes faster with online surveys. Responses can be collected instantaneously, allowing for rapid analysis.
- User-Friendly: SurveyMonkey offers easy-to-implement templates. This means that even those with minimal technical skills can create effective surveys.
- Real-Time Feedback: Researchers can track responses in real-time. This instantaneity can be crucial for making timely decisions.
Incorporating online surveys into the data collection strategy transforms how organizations approach their research. The confluence of SurveyMonkey's functionalities and SPSS's analytical capabilities creates a robust framework for businesses seeking to enhance their operational efficiency.
Integrating SurveyMonkey with SPSS
The integration of SurveyMonkey with SPSS is a crucial step in streamlining survey data analysis. Both platforms serve unique purposes: SurveyMonkey excels in data collection while SPSS is a powerful tool for data analysis. Combining these tools effectively enables researchers to harness the strengths of each platform, leading to more insightful outcomes.
By integrating SurveyMonkey with SPSS, users gain access to a robust framework for handling and interpreting survey data. The process helps in reducing manual data entry errors, saving time, and ensuring that data is accurately represented. Moreover, this integration allows users to leverage sophisticated statistical techniques in SPSS after collecting data seamlessly from SurveyMonkey.
Exporting Data from SurveyMonkey
Once the survey is completed, the next step involves exporting the data from SurveyMonkey. This process is generally straightforward and can be accomplished by following these steps:
- Log into your SurveyMonkey account.
- Navigate to the "My Surveys" section and select the survey you want to analyze.
- Click on the "Analyze Results" tab.
- Choose the option to export data. You can select formats that suit your needs, although the most common choice is CSV.
- Download the file to your local system.
This exported CSV file will contain all the responses collected, formatted in a way that is compatible with SPSS. It is essential to ensure that the data is cleaned and de-duplicated before moving to the next step. Accurate exporting is fundamental to maintaining data integrity.
Importing Data into SPSS
After successfully exporting the data, the next task is to import it into SPSS. This step is equally critical and involves several considerations:
- Open SPSS and create a new data file.
- Go to the "File" menu, then select "Import Data" and choose the "Text Data" option for CSV files.
- Locate the downloaded CSV file from SurveyMonkey and click "Open".
- Follow the prompts in the Text Import Wizard. Here, it’s important to check column settings and variable types to ensure smooth data integration.
- Once done, you can proceed with your data analysis.
It is prudent to check the imported data for any inconsistencies or anomalies that might have arisen during the export-import process. This meticulous approach prevents potential errors in analysis, ensuring reliable insights.
Integrating SurveyMonkey with SPSS offers immense benefits. It enables a seamless transition of data from collection to analysis, allowing businesses and researchers to focus on deriving insights rather than just managing data. The efficiency gained through this integration can empower organizations to make informed decisions quickly.
Data Analysis: Utilizing SPSS Tools
Data analysis is a critical step in any research process. Utilizing SPSS tools can greatly enhance the insights derived from survey data collected through SurveyMonkey. SPSS, or Statistical Package for the Social Sciences, offers a robust suite of analytical capabilities that empower businesses to uncover patterns, trends, and relationships in their data. By leveraging SPSS, organizations can move from mere data collection to meaningful interpretation, transforming raw numbers into actionable insights. This section will delve into two main areas: Descriptive Statistics and Inferential Statistical Tests.
Descriptive Statistics in SPSS
Descriptive statistics serve as the foundation for understanding data. These statistics help summarize data sets in a meaningful way. In SPSS, users can compute measures like mean, median, mode, variance, and standard deviation. These metrics provide a snapshot of the data, allowing researchers to identify central tendencies and variability.
- Mean provides an average, offering insights into what is typical in a data set.
- Median indicates the middle value, which is particularly useful in understanding distributions skewed by outliers.
- Mode shows the most frequently occurring value, useful for identifying common responses.
- Variance and Standard Deviation measure how much individual values differ from the mean, revealing the data's spread.
Using SPSS, one can create visual representations, such as histograms, bar charts, and box plots, to illustrate these statistics. This visual context aids in interpreting complex data, making it easier for stakeholders to grasp essential findings.
Conducting Inferential Statistical Tests
Once descriptive statistics are understood, researchers often wish to make inferences beyond their immediate data sets. Inferential statistical tests allow businesses to draw conclusions about a population based on sample data. SPSS facilitates numerous tests that can uncover significant differences or relationships.
Some common inferential tests include:
- T-tests: Compare means between two groups (e.g., survey respondents versus non-respondents).
- Chi-square tests: Assess the association between categorical variables.
- ANOVA (Analysis of Variance): Examine differences among three or more group means simultaneously.
- Regression Analysis: Explore relationships between variables, determining the strength and direction of these relationships.
Utilizing these tests correctly can help businesses validate hypotheses, enabling more strategic decision-making based on sound evidence.
Remember: The validity of results from inferential tests depends significantly on the quality of data collected and the appropriateness of the chosen tests based on data type. Therefore, it’s essential to understand the underlying assumptions of each test before application.
Interpreting Survey Results
Interpreting survey results is a crucial phase in the research process, forming the bridge between data collection and actionable insights. Within the context of SurveyMonkey and SPSS, this step involves analyzing the data gathered from surveys to draw meaningful conclusions and direct future strategies. This process not only aids businesses in making informed decisions but also enhances their operational effectiveness. The relevance of this topic lies in its capacity to transform raw data into digestible insights that can drive organizational change and improve product offerings.
Key Metrics to Analyze
When interpreting survey results, certain metrics stand out as particularly significant. Understanding these metrics can guide analysts in their assessments and help them connect the data to business objectives. Here are some key metrics to consider:
- Response Rate: This indicates how many people completed the survey compared to how many received it. A high response rate often signifies that the survey was relevant and engaging, while a low rate might point to issues in the survey design.
- Net Promoter Score (NPS): This metric gauges customer loyalty and satisfaction by asking respondents how likely they are to recommend a product or service. Analyzing NPS can provide businesses with a clear view of their customer relationships.
- Mean and Median Scores: These statistics provide insight into the overall sentiment of survey participants. Whereas the mean gives an average response, the median helps to understand the central tendency, dampening the impact of outliers.
- Frequency Distribution: This shows how often each response was given, helping to identify patterns or trends in preferences across a demographic.
Analyzing these metrics allows organizations to not only assess satisfaction but also to identify possible areas for improvement, directly influencing strategic decisions.
Visualizing Data in SPSS
Data visualization is an essential element of data analysis, especially when working with complex datasets. SPSS offers powerful graphical tools that can turn data points into clear and informative visuals. This process aids in comprehending patterns that may not be evident in raw data. Here are some strategies for effective data visualization in SPSS:
- Bar Charts and Histograms: Useful for showcasing frequency distributions, these visuals can highlight the differences in survey responses across different categories.
- Pie Charts: Though sometimes criticized for oversimplification, pie charts can effectively illustrate proportional data, helping stakeholders quickly see the distribution of responses.
- Box Plots: These plots display the median, quartiles, and potential outliers in datasets, providing a clear summary of data distribution and variability.
- Scatter Plots: Ideal for showing relationships between two variables, scatter plots can help uncover correlations, adding depth to the analysis.
In utilizing these visualization techniques, businesses can enhance their understanding of complex survey data and present findings in an accessible manner for decision-makers.
Effective visualization can lead to clearer insights and more directed actions from data analysis.
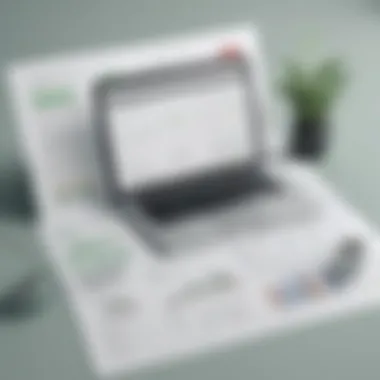
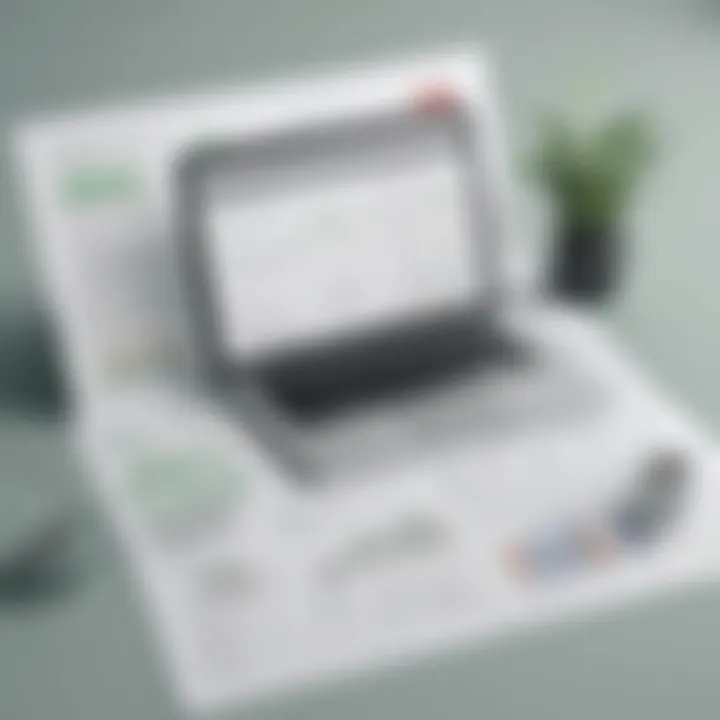
Practical Applications Across Industries
Understanding the practical applications of combining SurveyMonkey and SPSS has significant implications for how businesses and organizations harness data. The integration of these two platforms goes beyond mere data collection and analysis; it enables organizations to transform raw data into actionable insights. The benefits are manifold, making this combination vital for diverse industries.
Market Research and Consumer Insights
Market research is essential for any organization wanting to understand its audience. SurveyMonkey excels in generating surveys quickly and efficiently. The platform provides a wide range of customization options to tailor each survey, ensuring it resonates well with participants. As businesses gather responses, they can leverage SPSS's analytical power to dissect the data. Through descriptive and inferential statistics, patterns emerge. This insight aids businesses in recognizing consumer behavior, preferences, and emerging trends.
Using SurveyMonkey to gather data combined with SPSS’s capabilities allows for deeper analysis such as segmentation and trend forecasting. Moreover, businesses can conduct A/B testing to measure the effectiveness of marketing campaigns. This analysis can inform strategic planning, leading to better-targeted advertisements and enhanced customer engagement. Overall, this synergy offers businesses the tools needed to make data-driven decisions and fine-tune their marketing strategies.
Employee Feedback and Organizational Assessment
Employee feedback is a critical aspect of organizational health. SurveyMonkey provides an easy-to-use interface for conducting employee satisfaction surveys. Questions can be designed to probe various dimensions of workplace culture, from job satisfaction to team dynamics. Anonymity ensures that employees feel safe to express their opinions, leading to more honest feedback.
Post data collection, SPSS plays a crucial role. Organizations can analyze trends over time and measure the impact of initiatives aimed at improving workplace culture. For instance, understanding the correlation between employee satisfaction scores and productivity can help management identify areas for improvement.
Furthermore, SPSS offers tools to visualize data, which can be crucial when sharing findings with stakeholders. Effective communication of survey results can aid in fostering a culture of transparency and engagement within the organization.
By harnessing these tools, organizations not only mold their internal practices based on feedback but also enhance the overall employee experience. Integrating SurveyMonkey and SPSS allows for a robust framework for evaluation and ongoing development within any team.
Best Practices for Effective Survey Design
Effective survey design is crucial for obtaining actionable data. By following best practices, businesses can maximize response rates and ensure that collected data is reliable and valid. Attention to survey design impacts the overall quality of insights derived from the research process. This section delves into two critical elements: crafting clear and concise questions and utilizing question types effectively.
Crafting Clear and Concise Questions
When constructing a survey, clarity is paramount. Questions should be simple, direct, and free of jargon or complex phrasing. A participant’s understanding of a question directly correlates to the accuracy of their response.
Some key points to consider include:
- Be Direct: Avoid vague language. Instead of asking, "How do you feel about our service?" specify what aspect you want feedback on, such as, "How would you rate the response time of our customer support?"
- Limit Questions: Each question should address a single idea. Asking multiple things in one question can confuse respondents and lead to inaccurate data. For example, instead of asking, "Did you find our website easy to navigate and visually appealing?" focus on one aspect per question.
- Use Neutral Language: Be cautious of wording that might lead respondents toward a particular answer. For example, instead of asking, "How much do you love our product?" it is better to ask, "How satisfied are you with our product?"
"The clarity of survey questions shapes the quality of data collected. Misunderstood questions can yield misleading results, which undercuts the survey's purpose."
Utilizing Question Types Effectively
Surveys can feature various question types, each having its own strengths and uses. Here’s how to use them effectively:
- Closed-ended Questions: Good for quantitative analysis, these limit responses and make data analysis straightforward. Options can include multiple-choice, rating scales, or yes/no responses.
- Open-ended Questions: Useful for qualitative insights, allowing respondents to express their thoughts freely. However, these can be challenging to analyze, so they should be used selectively. For example, combining a closed-ended question about satisfaction with an open-ended follow-up for suggestions can provide depth.
- Matrix Questions: These allow respondents to evaluate multiple items on a single scale. They save space and facilitate easy comparisons. For instance, they can be used to assess various attributes of a service in one go.
Each question type should align with the research goals. Consider the audience as well; for some groups, visual elements or more complex question formats might yield better engagement. Ensuring a thoughtful selection of question types can lead to higher completeness and relevance of the data collected.
By adhering to these best practices in survey design, businesses can significantly enhance the effectiveness of their data collection efforts, paving the way for clearer insights and informed decision-making.
Common Challenges in Data Analysis
Data analysis is a critical component of the research process, particularly for organizations leveraging tools like SurveyMonkey and SPSS. However, practitioners often encounter significant challenges that can hinder the accuracy and effectiveness of their findings. Understanding these challenges is vital for small to medium-sized businesses, entrepreneurs, and IT professionals who depend on reliable data for decision-making.
Survey data can often present complexity that complicates straight analysis. Researchers must navigate the nuances of data integrity, representativity, and analytical precision. Addressing these challenges early in the data analysis process not only improves outcomes but also builds trust in the results.
Handling Missing Data
One common issue in survey data analysis is missing data. This can occur for various reasons, such as respondents skipping questions or technical errors during data collection. Handling missing data is essential because it can skew results and reduce the validity of conclusions drawn from the analysis.
There are several methods to address this issue:
- Deletion: Removing data entries with missing values can simplify analysis. However, this may lead to bias, especially if the missing data is not random.
- Imputation: Filling in missing data with estimated values based on other available information can help retain sample size. Techniques such as mean substitution or regression imputation can be useful.
- Weighting: Adjusting the analysis to give more emphasis to underrepresented groups can help counterbalance the impact of missing data.
Choosing the right method requires careful consideration of the nature of the missing data and its potential impact on research outcomes. It often requires a delicate balance between maintaining the dataset's integrity and achieving reliable results.
Addressing Bias in Survey Responses
Bias in survey responses is another challenge that can distort data and lead to incorrect conclusions. Bias can stem from various sources, including poorly worded questions, leading or ambiguous response options, and the demographic characteristics of respondents.
To mitigate bias, consider the following strategies:
- Question Design: Formulate questions that are clear and neutral. Avoid language that could suggest a preferred response.
- Pre-testing: Conduct pilot surveys to identify and correct potential biases before launching the full survey.
- Diversifying Respondent Pool: Ensuring that your respondent demographics accurately reflect the population of interest can help produce more representative data.
Ultimately, identifying and addressing bias is critical for enhancing the credibility of survey research. By considering these elements, businesses and researchers can improve the reliability of their analyses and the actions they take based on the findings.
"Efforts to understand and address biases enhance the trustworthiness of data analysis outcomes."
Case Studies: Successful Implementations
Case studies provide real-world insights that illustrate the practical applications of integrating SurveyMonkey and SPSS. By examining successful implementations, organizations can understand how to leverage these tools effectively.
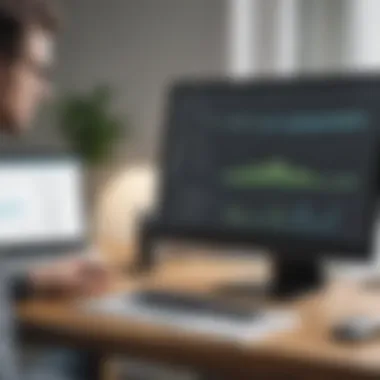
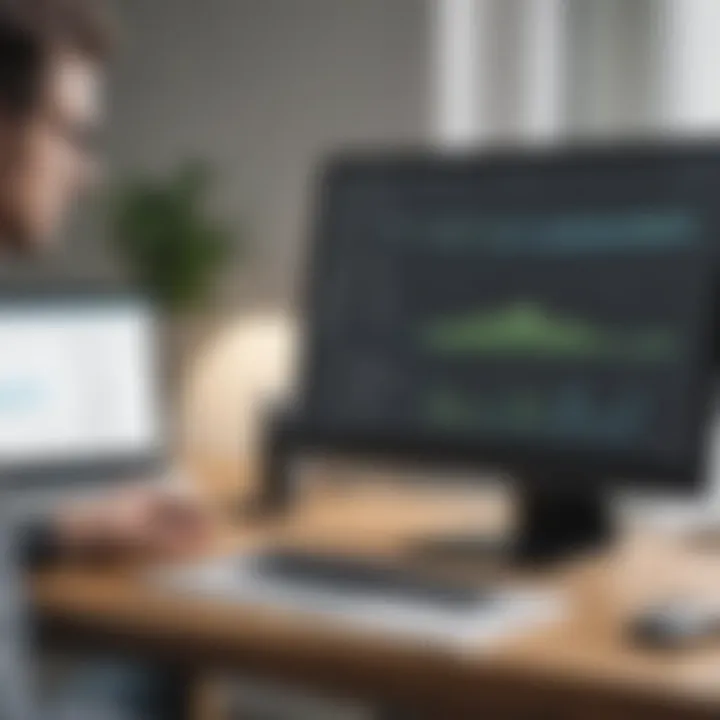
These case studies not only highlight the strengths of both platforms but also showcase the methodologies that led to successful results. They serve as tangible examples for small to medium-sized businesses and entrepreneurs who may be considering similar strategies. Understanding these implementations can guide important decisions, especially in areas of data collection and analysis.
Key benefits of examining case studies include:
- Practical Insights: Real-life examples illustrate theoretical concepts.
- Learning from Mistakes: Case studies often reveal common pitfalls and how to avoid them.
- Industry Relevance: Different sectors may face unique challenges and insights, offering a broader perspective.
- Inspiration and Innovation: Organizations can spark new ideas to enhance their research strategies.
Case Study One: Retail Sector Insights
A major retail company utilized SurveyMonkey to gather customer feedback on their shopping experience. They designed surveys to gauge customer satisfaction, product preferences, and shopping habits. The data collected was then exported to SPSS for detailed analysis.
Using SPSS, the company applied descriptive statistics to summarize the data. They calculated mean satisfaction levels and identified trends in customer preferences across different demographics. By conducting inferential tests, they could confirm whether observed differences in satisfaction were statistically significant.
This analysis revealed some surprising insights:
- Most satisfied customers were those who received personalized marketing.
- Younger customers preferred a seamless online shopping experience.
- Pricing strategies varied in effectiveness across regions.
These findings enabled the company to better tailor their marketing strategies and improve customer retention rates. They implemented changes based on quantitative feedback, thus boosting both customer satisfaction and sales.
Case Study Two: Education Sector Feedback
In the education sector, a university sought feedback from students regarding an online course. To do this, they employed SurveyMonkey to create necessary surveys. The surveys targeted various aspects such as course content, instructor effectiveness, and overall student satisfaction.
After collecting the data, the university imported it into SPSS to analyze the results. They used visualization tools within SPSS to present findings clearly, which helped stakeholders easily understand student perceptions.
Through analysis, it became evident that students favored interactive components in courses. The university also discovered a significant gap in satisfaction among different student demographics. Some key insights included:
- Students in smaller classes felt more engaged compared to larger groups.
- The use of multimedia resources positively influenced course evaluations.
- Lack of communication from instructors negatively impacted student satisfaction.
These insights prompted significant changes in course design and teaching style. By addressing the identified issues, the university enhanced the overall student experience and improved course ratings.
"Successful data analysis can turn survey insights into action. Implementing changes based on real feedback leads to improvement."
By studying implementations in both cases, organizations can garner practical approaches for their specific sectors. Understanding the integration of SurveyMonkey and SPSS through these examples enhances both data analysis skills and strategic direction.
Future Trends in Data Collection and Analysis
The landscape of data collection and analysis is undergoing significant transformations. Understanding these trends is essential for businesses aiming to leverage data effectively. Small to medium-sized businesses and entrepreneurs, in particular, can benefit significantly from staying ahead of these trends. By adapting to new methodologies, they can enhance their research capabilities, improve decision-making, and generate better insights.
Emerging Technologies in Research
Technological advancements continually shape how data is collected and analyzed. Several emerging technologies have gained traction in research settings. These technologies include:
- Cloud Computing: Facilitates efficient data storage and accessibility. Researchers can collect and analyze large data sets without heavy upfront investments in infrastructure.
- Mobile Data Collection: Mobile surveys and applications allow for real-time data capture. This is particularly advantageous for conducting field research or gathering feedback quickly.
- Wearable Devices: Wearables generate vast amounts of data, providing rich insights into user behavior and health trends. This can enhance research in various sectors including healthcare and marketing.
These tools create opportunities for more accurate data collection and deeper analysis. The integration of these technologies can considerably enhance the quality and speed of research outputs.
The Role of Artificial Intelligence in Data Analysis
Artificial Intelligence (AI) has emerged as a game-changer in data analysis. It offers several advantages that make it a powerful ally for researchers using SurveyMonkey and SPSS. Here are some significant contributions of AI in this domain:
- Automating Data Processing: AI can automate repetitive tasks involved in data cleaning and preparation. This reduces the time researchers spend on mundane processes and allows them to focus on analysis.
- Predictive Analytics: AI algorithms can analyze data and identify trends, enabling businesses to forecast outcomes. This predictive capability supports proactive decision-making.
- Natural Language Processing: AI-driven tools can analyze qualitative data from open-ended survey responses. This helps in gathering insights from unstructured data and enhances understanding of respondent sentiments.
"The convergence of advanced technology and data analysis promises not only efficiency but also enriched insights that drive strategic growth."
Staying abreast of these trends is vital for those involved in data research and analysis.
The End
In the realm of data analysis, the topic of concluding insights holds significant weight. This article has extensively examined how to effectively merge the capabilities of SurveyMonkey and SPSS, providing a robust framework for data collection and analysis. The importance of drawing clear conclusions from the data gathered cannot be understated.
Summarizing Key Insights
Conclusions enable businesses to distill complex data into actionable insights. Here are several key elements to consider:
- Data Interpretation: Leveraging SPSS tools allows for a nuanced understanding of survey results. It is crucial to interpret the findings beyond mere numbers, looking at trends and patterns.
- Actionable Outcomes: The goal of using SurveyMonkey in conjunction with SPSS is to extract insights that can inform decision-making. Small to medium-sized businesses can capitalize on these insights for strategic planning.
- Best Practices: Integrating both platforms enhances the reliability of results. Clear questions in SurveyMonkey lead to cleaner data. Following best practices in survey design ensures validity and relevance.
- Addressing Challenges: Throughout data analysis, challenges will arise, such as missing data or biased responses. It is essential to recognize and navigate these issues for credible conclusions.
- Future Considerations: As technology evolves, so do the methodologies for data collection and analysis. Keeping abreast of trends in research technology, such as artificial intelligence, fosters a forward-thinking approach.
This integration is not merely a checklist; it represents a paradigmatic shift in how businesses can utilize survey data. The ability to draw insightful conclusions from SurveyMonkey and SPSS is a vital component of modern research practices, especially for organizations aiming for growth and innovation.
Importance of References
In any analytical work, references serve multiple essential functions. They provide a foundation for the arguments made and enhance the reader's confidence in the conclusions drawn. When discussing methodologies in data collection and analysis, like those provided by SurveyMonkey and SPSS, referencing key texts and studies lends strength to the claims about effectiveness and reliability.
Benefits of Citing Sources
- Credibility: Citing established research and methodologies elevates the piece. Readers engage more thoroughly with policies backed by recognized studies or authorities.
- Depth of Insight: References open pathways to further reading and understanding. They guide readers to explore the tools and techniques utilized in the article more deeply.
- Accountability: Proper citation reflects the diligence and integrity of the author. It shows respect for intellectual property and encourages ethical research practices.
Considerations for Using References
When including references, several considerations are important:
- Relevance: Ensure that the sources directly relate to SurveyMonkey and SPSS functionalities.
- Recency: Use recent studies and data to provide an accurate and contemporary viewpoint. The field of data analysis evolves quickly, and outdated references could mislead.
- Diversity of Sources: Include a mix of articles, journals, and user forums to give a balanced narrative. For instance, academic insights may be enhanced with practical user experiences shared on platforms like Reddit or Facebook.
The article draws upon these principles as we analyze the strengths and applications of SurveyMonkey and SPSS together. By effectively integrating references, readers can appreciate not only the current capabilities of each tool but also their historical and ongoing place in data analysis.