Exploring Top NLP Companies for Business Success
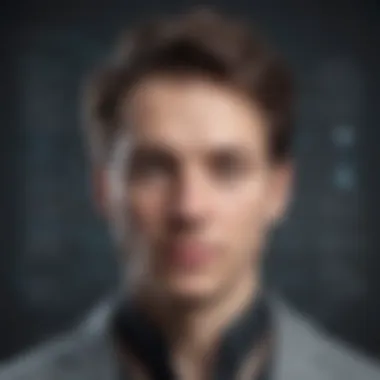
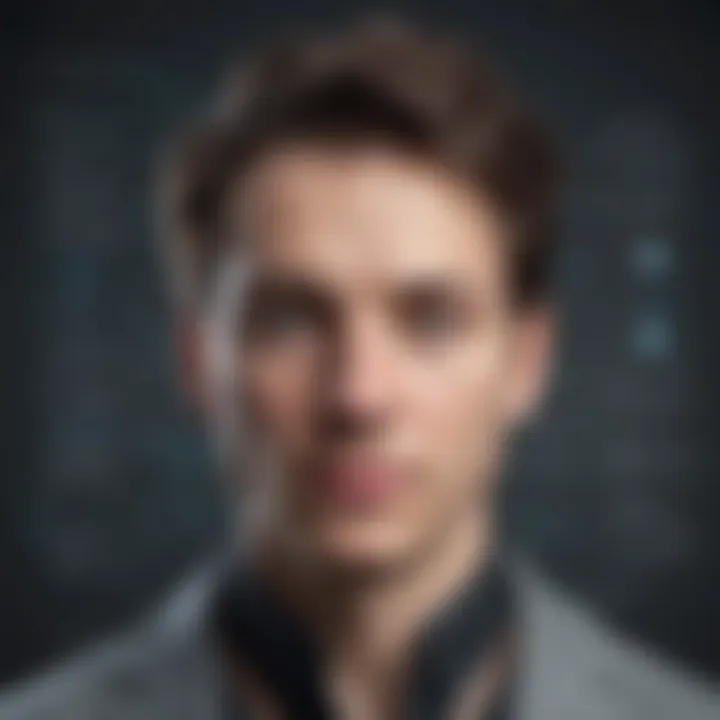
Intro
The rise of natural language processing (NLP) technology is influencing how businesses operate. As companies seek to enhance their efficiency, they are increasingly turning to NLP solutions. This shift is not limited to large corporations; small and medium-sized enterprises are also recognizing the value of these technologies.
In this article, we explore leading NLP companies, their offerings, and how they are shaping the future of business. Understanding these players is critical for informed decision-making in selecting the appropriate NLP solution. Readers will gain insights into the strengths of each company, their technologies, and the applications available to address distinct business needs.
Software Overview
Natural language processing software serves a crucial purpose in analyzing, understanding, and generating human language. By leveraging machine learning algorithms and linguistic rules, NLP solutions assist organizations in processing volumes of text data efficiently. This enhances workflows and improves accuracy in communication and understanding.
Purpose of the Software
The primary aim of NLP software is to facilitate smoother interaction between humans and machines. This includes automating tasks such as sentiment analysis, text classification, and chatbots for customer support. Businesses utilize this technology to:
- Enhance customer engagement
- Automate repetitive tasks
- Improve content categorization
- Enable data-driven decision-making
Key Features
NLP software includes various features tailored to address the specific needs of businesses. Some notable features are:
- Entity Recognition: Identifies entities such as names and organizations, enabling better data extraction.
- Sentiment Analysis: Evaluates the sentiment behind texts, providing valuable insights into customer opinions.
- Text Summarization: Condenses large bodies of text, making information easier to digest.
- Translation Services: Implements real-time translation, supporting global communication.
Comparison with Competitors
When evaluating NLP companies, it is vital to perform a feature-by-feature analysis. This allows businesses to determine which NLP solution meets their particular requirements best.
Feature-by-Feature Analysis
In the competitive landscape, companies like Google Cloud Natural Language, Microsoft Azure Text Analytics, and IBM Watson NLP stand out. Each offers unique features, such as:
- Google Cloud Natural Language: Focuses on syntax analysis and powerful entity recognition capabilities.
- Microsoft Azure Text Analytics: Specializes in sentiment analysis and language detection, appealing to marketing teams or customer service departments.
- IBM Watson NLP: Provides advanced language algorithms for complex text analysis.
Each of these solutions has direct implications for productivity and accuracy in business settings.
Pricing Comparison
Pricing is another crucial factor when selecting an NLP solution. For small to medium-sized businesses, understanding costs is essential. Here is a general comparison:
- Google Cloud Natural Language: Charges based on usage with a free tier for developers.
- Microsoft Azure Text Analytics: Offers a pay-as-you-go model, appealing for fluctuating needs.
- IBM Watson NLP: Subscription-based pricing, best suited for long-term projects.
Ultimately, the choice of NLP solution should align with specific business goals and budget considerations.
Preface to Natural Language Processing
Natural Language Processing (NLP) stands at the intersection of linguistics and artificial intelligence. This field equips machines with the ability to understand, interpret, and respond to human language in a manner that is both meaningful and contextually appropriate. The significance of NLP extends beyond mere language comprehension; it encompasses a variety of tasks, including language generation, sentiment analysis, and machine translation.
NLP has gained prominence due to the increasing reliance of businesses on data-driven decisions. As organizations accumulate vast amounts of text-based data from customer feedback, emails, and social media, extracting actionable insights becomes essential. Therefore, understanding NLP is not just an academic pursuit but a practical undertaking that can enhance business operations and customer engagement.
In this article, we will explore various aspects of NLP, focusing on its applications within industry, the leading companies shaping the technology, and how small to medium-sized enterprises can leverage these solutions. By grasping the fundamentals of NLP and its relevance in today's data-centric world, businesses can strategically enhance their processes and adapt to evolving customer needs.
Understanding NLP
Natural Language Processing is a critical subfield of artificial intelligence that focuses on the interaction between computers and humans through natural language. It incorporates several disciplines, including computer science, linguistics, and cognitive psychology. The goal of NLP is to enable machines to process and analyze large amounts of language data effectively.
Some fundamental tasks within NLP include:
- Text processing: Cleaning and preparing textual data for analysis.
- Tokenization: Breaking down text into smaller units, such as words or phrases.
- Part-of-speech tagging: Identifying the grammatical roles of words in sentences.
- Named entity recognition: Recognizing and classifying key elements in the text, such as names, dates, and locations.
Technology underpins these tasks. Algorithms and models, including machine learning and deep learning, play crucial roles in enhancing NLP's capabilities. As these technologies develop, the accuracy and efficiency of NLP systems continually improve.
Importance of NLP in Business
The integration of NLP into business operations is transforming how organizations interact with their clients. With customers increasingly expecting personalized and rapid responses, NLP can bridge the gap effectively. Here are several reasons why NLP is essential for modern businesses:
- Improved customer service: Chatbots and virtual assistants, powered by NLP, provide immediate assistance, addressing user inquiries without human intervention.
- Enhanced data analysis: Businesses can extract sentiment and themes from customer feedback, allowing for data-driven decision-making.
- Automation of repetitive tasks: NLP can automate processes like data entry and report generation, freeing up human resources for higher-level tasks.
- Competitive advantage: Companies leveraging NLP technologies can respond faster to market changes and customer needs, positioning themselves ahead of competitors.
In summary, understanding and implementing NLP in business is more than an advantageous option; it is becoming necessary in today's fast-paced digital landscape. As we delve deeper into the specifics of NLP companies throughout this article, we aim to provide a comprehensive guide to help organizations navigate this evolving technological space.
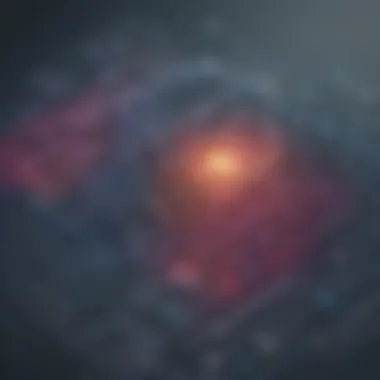
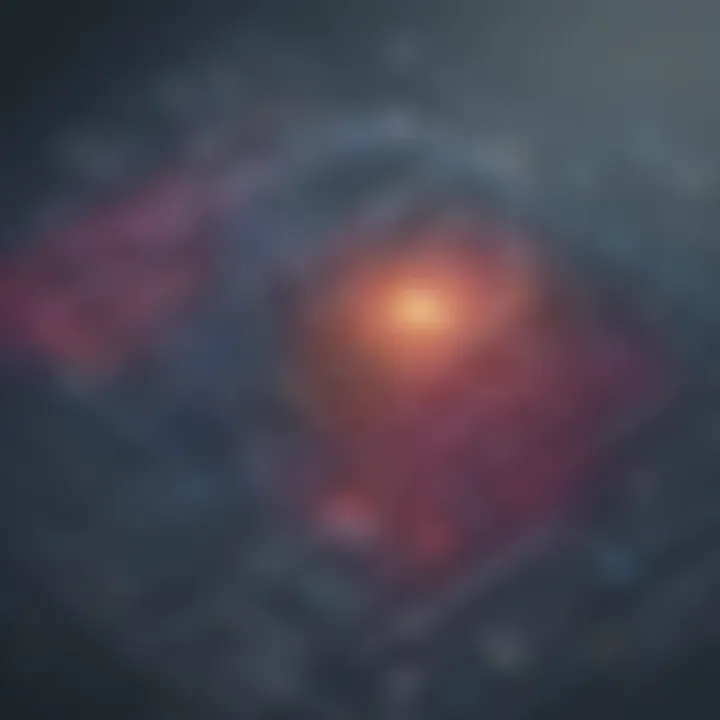
Criteria for Evaluating NLP Companies
Evaluating NLP companies is critical for businesses that wish to implement natural language processing solutions. The right company can significantly enhance operational efficiency and create a competitive advantage. Therefore, understanding what to look for in an NLP provider is essential. Key criteria include technology and innovation, market presence and reputation, as well as customer support and service. These elements shape the quality and reliability of NLP solutions.
Technology and Innovation
When assessing an NLP company, technology and innovation are prime factors. Businesses should look at the tools and algorithms that a company uses. For example, companies using state-of-the-art machine learning and deep learning methods are likely to provide better performance and accuracy. Innovation also plays a role here. Companies that continuously invest in research and development typically offer cutting-edge solutions. This ensures that their offerings remain relevant and effective, catering to the evolving needs of businesses. It is also important to note that proprietary models can give companies a significant edge, as they can tailor solutions more specifically to user demands.
Market Presence and Reputation
Market presence and reputation provide insights into how a company is perceived in the industry. Established companies often have a track record of successful implementations, which can help mitigate risks associated with new partnerships. Checking user reviews and case studies can paint a clearer picture. Furthermore, an NLP firm's presence in key industry events or partnerships with respected organizations can demonstrate credibility. This aspect often indicates how well the company understands market needs and trends, making it easier for potential clients to trust in their capabilities.
Customer Support and Service
Customer support is a vital consideration when selecting an NLP company. Implementation of NLP solutions can involve complexities, and having effective support is essential for minimizing disruptions. Companies that offer robust training programs can help clients maximize the utility of their products. Moreover, accessible customer service can aid in swiftly resolving issues that may arise post-deployment. Ongoing support options, such as software updates and maintenance, also influence the long-term viability of the partnership. Therefore, evaluating customer support services should not be overlooked in the decision-making process.
Understanding these criteria ensures that businesses can make informed decisions when choosing an NLP provider, aligning their needs with the strengths of the offered solutions.
Overview of Leading NLP Companies
The landscape of natural language processing (NLP) companies plays a crucial role in the evolution of technology solutions for numerous sectors. Understanding the strengths and capabilities of leading businesses in this field can help organizations make informed decisions about which technology to adopt. The overview also addresses some specific benefits, such as improving operational processes and enhancing communication with customers. Companies across various industries are integrating NLP solutions to keep pace with technological advancements and to enhance user experiences.
Company A: Profile and Offerings
Key Technologies
Company A has developed a robust suite of NLP tools that leverage advanced machine learning algorithms. This embrace of cutting-edge technology ensures accuracy and efficiency in text analysis and language understanding. A particular strength of its NLP suite lies in its emphasis on natural language generation. Users find this beneficial as it streamlines content creation processes significantly. The unique feature of Company A's technology is its ability to adapt to various languages and dialects, making it a versatile choice for organizations operating in multiple markets.
Industry Applications
The services provided by Company A span numerous industries, including e-commerce, healthcare, and finance. This versatility indicates its strong market presence, as the tools are designed to enhance customer interaction and data analysis. For instance, in e-commerce, the NLP solutions facilitate personalized shopping experiences through customer insights gathered from interactions. The remarkable feature of these applications is their scalability, allowing businesses of all sizes to implement effective solutions without excessive overhead.
Market Position
Company A has established itself as a leader in the NLP domain. Its reputation is built on continuous innovation and a strong client base. The key characteristic contributing to its market position is the ability to deliver reliable solutions. This reliability makes it a popular choice for companies seeking consistent performance in NLP tasks. However, focusing primarily on corporate clients could present a disadvantage for smaller businesses that may find costs prohibitive.
Company B: Profile and Offerings
Key Technologies
Company B emphasizes user-friendly NLP technology that integrates seamlessly with existing systems. Its unique selling proposition lies in its accessibility and ease of use. The platform is designed to be intuitive, which benefits IT professionals by reducing the learning curve associated with new technology. Furthermore, its integration with data analytics tools enhances decision-making capabilities across the board.
Industry Applications
In financial services, Company B applies its NLP technologies to risk assessment and fraud detection. The NLP tools analyze customer communication to identify potential threats in real time. This feature is critical as it can provide organizations with immediate alerts, enhancing security protocols. However, the reliance on large volumes of data can create challenges in terms of data management and privacy concerns.
Market Position
Company B enjoys a solid reputation due to its focus on customer-centric solutions. Its market position benefits from strategic partnerships with leading tech firms, which enhance credibility. A potential challenge for Company B lies in competition from newer firms that may offer lower prices or niche solutions.
Company C: Profile and Offerings
Key Technologies
Company C's technology is built on deep learning techniques, allowing the system to recognize context and subtleties in language use. This capability is particularly useful in applications requiring sentiment analysis. The standout characteristic is its high accuracy in understanding user intent, making it a preferred choice for companies focused on enhancing customer satisfaction. However, implementing such complex technologies can require significant resources.
Industry Applications
In healthcare, Company C implements NLP to streamline patient records management. The technology extracts relevant data from unstructured text, improving efficiency in care delivery. This unique application showcases the transformative nature of NLP in environments that require precision. However, the challenge remains in ensuring the accuracy of information sourced from various document types.
Market Position
Company C holds a strategic position in the healthcare sector, aided by its specialized tools for medical language processing. It is recognized not only for its technological leadership but also for its educational initiatives aimed at user training. Yet, this research-focused approach might limit its adaptability to the fast-paced changes typical in the broader tech market.
Company D: Profile and Offerings
Key Technologies
Company D specializes in creating hybrid NLP solutions that incorporate both rule-based and machine learning algorithms. This dual approach allows for more tailored applications while addressing diverse user needs. The unique aspect of its technology is the flexibility it provides, allowing companies to customize their NLP tools to match specific operational requirements.
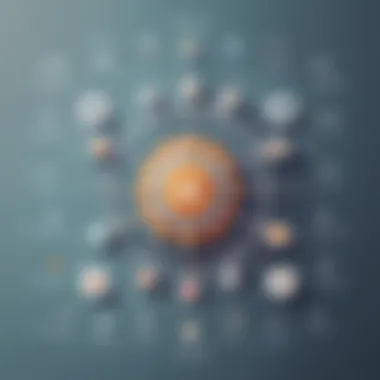
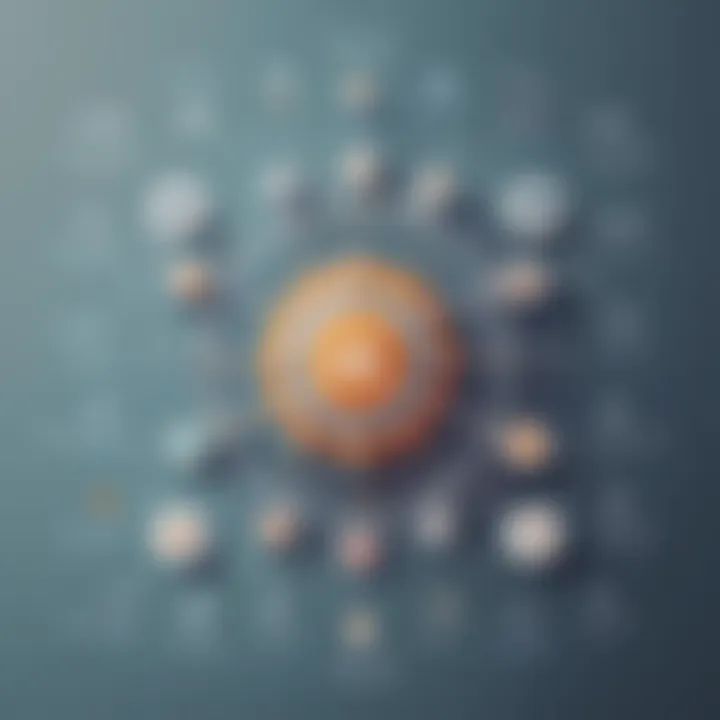
Industry Applications
Company Dโs tools are versatile across industries such as telecommunications and entertainment. Their NLP solutions aid in voice recognition systems, enhancing user interactions significantly. The notable feature is the adaptability to varied channels like chat, voice, and text, which is critical for organizations aiming for a multichannel strategy. However, this complexity requires substantial investment in both time and training.
Market Position
Company D is recognized for its commitment to innovation and customization. Its market position is strong, especially among clients looking for tailored solutions. But the potential downside is its higher price point, which can make it less accessible for small businesses.
In an era where every interaction counts, selecting the right NLP partner is not merely strategic; it's essential.
Comparative Analysis of NLP Technologies
The comparative analysis of NLP technologies is essential for understanding how different approaches influence the effectiveness of natural language processing solutions. This section delves into three primary methodologies: machine learning approaches, deep learning techniques, and traditional rule-based systems. Each of these methods you will be able to see have distinct characteristics, advantages, and limitations. Knowing these details helps businesses and professionals select the right technology to meet their needs effectively.
Machine Learning Approaches
Machine learning approaches are the backbone of modern NLP technologies. They rely on algorithms that learn from data, enabling systems to improve their performance over time. These techniques can process vast amounts of text efficiently, learning language patterns and context.
One significant benefit of machine learning approaches is their adaptability. They can be trained on specific datasets, which allows for tailored applications in various industries. For example, a company in the e-commerce sector can use tailored algorithms to analyze reviews, gaining insights into customer sentiment.
However, there are considerations to keep in mind. The performance heavily relies on the quality and volume of the training data. If the dataset is biased or limited, it can lead to poor results. Therefore, businesses should ensure they have comprehensive datasets that reflect diverse language use.
Deep Learning Techniques
Deep learning techniques represent a more advanced subset of machine learning, utilizing neural networks to model complex relationships within data. This approach is particularly powerful for handling unstructured data like text.
Deep learning methods, particularly with architectures like transformers, offer remarkable capabilities in understanding context and semantics. One of the prominent frameworks used in deep learning for NLP is BERT (Bidirectional Encoder Representations from Transformers), developed by Google. This framework can understand the context of a word based on surrounding words, which enhances the interpretation of language.
The advantages of deep learning are evident in the performance of tasks such as translation, summarization, and question-answering. However, these techniques often require significant computational resources and may not be practical for all businesses, especially small to medium-sized enterprises. Cost and infrastructure can pose challenges, so companies need to weigh their capabilities against their operational needs.
Traditional Rule-Based Systems
Traditional rule-based systems, while not as popular in recent years, still play a vital role in certain scenarios. These systems rely on predefined rules and linguistic structures to process language. They excel in applications where strict adherence to rules is necessary, such as legal or regulatory contexts.
An advantage of these systems is their interpretability. Businesses can easily understand why a particular decision or output was generated, making it valuable for domains that require transparency. Additionally, rule-based systems can be less resource-intensive than machine learning models, making them simpler to implement.
Nonetheless, traditional rule-based systems have limitations. They lack the flexibility of machine learning approaches and struggle with the nuances of natural language. Changes in language use or the introduction of new terms can render these systems less effective, as updating rules takes time and effort.
Understanding the strengths and weaknesses of NLP technologies equips businesses with the insights necessary to select the most suitable methodologies for their specific applications.
In summary, a comparative analysis of NLP technologies reveals that each approach has its distinct merits and challenges. By comprehending these differences, organizations can make informed decisions that align with their operational objectives and technological capabilities.
Industry Applications of NLP Technologies
NLP technologies have become essential tools across various industries, providing solutions that enhance operational efficiency and decision-making. By automating tasks such as text analysis, sentiment detection, and language translation, businesses can gain deeper insights into customer behavior and industry trends.
Implementing NLP solutions allows organizations to streamline workflows, reduce manual labor, and increase responsiveness to market changes. For small to medium-sized businesses, these applications can be particularly beneficial as they provide a competitive edge in todayโs data-driven environment.
NLP in Customer Service
Customer service is one of the areas where NLP shows remarkable impact. Businesses can utilize natural language processing to power chatbots and virtual assistants. Typcially, these AI-based solutions can easily handle frequently asked questions and perform basic troubleshooting.
Some advantages of applying NLP in customer service include:
- 24/7 Availability: Customers receive support at any time, enhancing satisfaction.
- Quick Response Times: Automated systems can reduce waiting time for responses.
- Data Collection: Gather insights from interactions, providing valuable information for improving services.
Moreover, NLP systems can detect customer sentiment from their messages. Businesses can then adjust their responses and strategies accordingly, addressing issues before they escalate.
NLP in Healthcare
In healthcare, NLP plays a crucial role in processing vast amounts of unstructured data. Medical records, research articles, and patient notes are just a few examples of the data that can be analyzed to enhance patient care.
Here are key applications:
- Clinical Documentation: Automating the creation of patient notes helps healthcare professionals focus more on patient care than admin tasks.
- Information Extraction: Extract crucial data from medical literature to aid in research and treatment planning.
- Patient Interaction: Chatbots can assist with scheduling appointments and answering health-related inquiries.
Using NLP in healthcare can lead to better patient outcomes and improved operational efficiencies, thereby making it easier to manage growing patient populations.
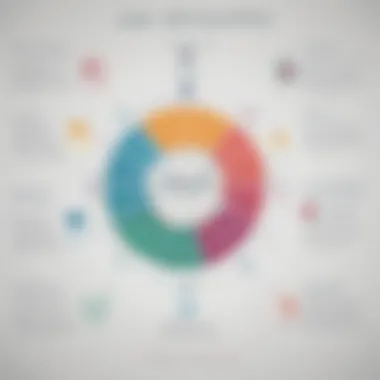
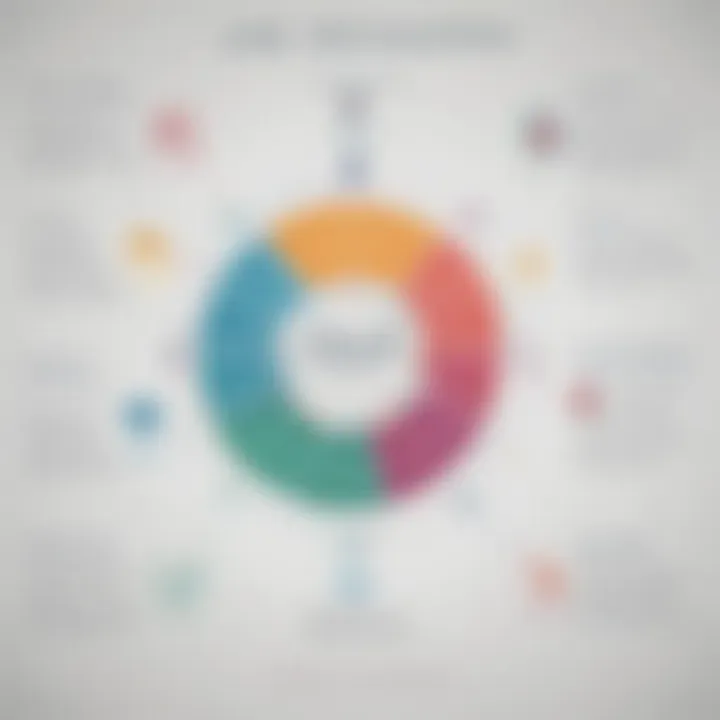
NLP in Finance
The finance sector also utilizes NLP for a variety of important applications. From fraud detection to sentiment analysis on financial news, NLP can be a game changer.
Key uses include:
- Risk Management: Analyzing reports and communications to assess risks in transactions or investments.
- Market Analysis: Understanding public sentiment towards certain stocks or commodities by processing social media and news feeds.
- Regulatory Compliance: Using NLP to ensure compliance with laws by analyzing communications and transactions.
Overall, financial institutions that leverage NLP can enhance customer experience and improve decision-making processes. More precisely, investing in NLP technologies can lead to better performance and higher trust with clients.
"Natural Language Processing is fundamentally changing how information is processed and understood in various industries."
With the increasing integration of NLP in these fields, companies should consider the unique advantages these technologies provide. As a result, the adoption of NLP is not merely an upgrade but a strategic move towards future readiness.
Challenges Faced by NLP Companies
As the sector of natural language processing (NLP) rapidly evolves, it faces numerous challenges that can hinder development and implementation. Identifying and understanding these challenges is crucial for small to medium-sized businesses, entrepreneurs, and IT professionals who are considering adopting these technologies. Each challenge not only affects the efficiency of NLP solutions but also poses concerns about the trustworthiness and scalability of these systems. Addressing these issues is vital for long-term success in the NLP industry.
Data Privacy Concerns
Data privacy is a significant issue for NLP companies. As these companies process vast amounts of text data, often containing sensitive information, the risk of data breaches becomes more prominent. Companies like OpenAI and Google invest heavily in infrastructure to protect user data. This investment is necessary to build trust with customers, particularly in sensitive industries like healthcare and finance.
Organizations must comply with regulations such as GDPR in Europe and CCPA in California, which govern how data is collected, stored, and processed. Failure to comply can lead to severe legal repercussions and a loss of reputation. Companies must implement effective solutions to anonymize data and ensure secure processing. Thus, a robust approach to data privacy contributes not only to compliance but also to customer confidence.
Bias in Training Data
Bias in training data remains a pressing issue in the NLP field. Algorithms learn from historical data inputs that may carry inherent biases. For instance, if a training dataset predominantly reflects certain demographics, the model may develop biased outcomes. This can severely impact sectors like recruitment or law enforcement, where decisions made by NLP systems can have real-life consequences.
Addressing bias requires systematic evaluation and diverse training data. Companies must carefully consider the datasets used in training. Regularly assessing model outputs for fairness is also crucial. Initiatives like the AI Fairness 360 toolkit can help organizations monitor and improve their systems. Investing in unbiased models not only promotes ethical standards but can also enhance user engagement and satisfaction.
Scalability Issues
Scalability poses another challenge for NLP companies. As the demand for NLP applications grows, companies must ensure their systems can handle increasing volumes of data and user requests without loss of performance. Scalability involves both infrastructure and model design. Some models may work well for smaller datasets but falter as data size expands.
Techniques like cloud computing offer solutions, allowing companies to scale their resources dynamically. However, challenges such as latency and cost variability come into play. Organizations need to develop systems that are not only efficient but also economically viable over time. By preparing infrastructure for future demands, companies can maintain their competitive edge while providing reliable services.
"In the fast-evolving landscape of NLP technologies, understanding and overcoming these challenges is crucial not only for business success but also for fostering a more equitable and secure technology environment."
Future Trends in NLP
Natural Language Processing is not static. It evolves rapidly in response to technological advancements and societal needs. A clear understanding of future trends in NLP can provide companies with insights that drive innovation, enhance competitive edge, and optimize operational efficiency. The importance of this topic lies in identifying which trends can be leveraged by businesses to improve their customer interactions and internal processes. Knowing these trends can guide small to medium-sized businesses in selecting the right NLP providers and technologies that align with their strategic objectives.
Advancements in AI Collaboration
The collaboration between AI and NLP is reaching new heights. Businesses are leveraging enhanced AI capabilities to interpret complex human language nuances. The emergence of models like OpenAI's GPT-3 and Google's BERT demonstrates how deep learning can better understand context, intention, and sentiment. Such advancements lead to more accurate responses in chatbots or virtual assistants.
Companies focusing on AI collaboration can design solutions that not only automate routine tasks but also learn from interactions to improve future performance. This adaptability can significantly reduce support costs and enhance user experience. For instance, integrating AI with platforms like Slack or Microsoft Teams can facilitate personal assistants that streamline workflow and maintain a conversational tone tailored to individual user needs.
Integration with Other Technologies
Integration of NLP with other emerging technologies promises to reshape business communications. When combined with fields like machine learning, big data analytics, and IoT, NLP can provide more robust solutions. For example, using IoT devices allows for real-time data capture. This data can be analyzed using NLP to create meaningful insights.
Moreover, integration with cloud computing enhances scalability and accessibility. Companies can store vast amounts of linguistic data, allowing for comprehensive analysis without significant upfront investments in infrastructure. One application of this is in customer feedback analysis. Businesses can gather customer insights from social media platforms, utilizing NLP to process this data into actionable reports.
"The future of NLP hinges on its ability to seamlessly integrate into diverse technological frameworks, addressing business needs effectively and efficiently."
In summary, staying abreast of future trends like AI collaboration and technology integration is vital for any business aiming to maximize the benefits of NLP. By recognizing how these trends take shape, small to medium-sized enterprises can make decisions that foster growth and innovation in a competitive marketplace.
The End
The conclusion of this article emphasizes the critical role of NLP companies in shaping the future of technology and business applications. This section serves as a final compendium of insights drawn from previous discussions. It reinforces the idea that understanding the strengths and weaknesses of each NLP provider is not just beneficial but essential for businesses aiming to harness the power of language processing.
Summary of Insights
In reviewing the various NLP companies discussed, several key themes emerge:
- Diversity of Applications: Each company presents unique offerings that cater to different industries, such as healthcare, finance, and customer service. Their specialized technologies can address specific challenges and enhance organizational efficiency.
- Technological Evolution: The landscape is rapidly evolving. Companies are increasingly adopting machine learning and deep learning techniques to refine their products. This transformation underscores the need for businesses to stay updated with the latest advancements.
- Importance of Market Presence: The reputation and market position of a company often reflect its capability and reliability. Market presence can serve as a metric for potential clients as they consider which NLP provider to partner with.
- Customization and Support: Effective customer support may differentiate one provider from another. Businesses should consider support services when selecting a vendor, as this can impact implementation and integration success.
Final Thoughts on Selecting an NLP Provider
Selecting an NLP provider is a multifaceted decision. Business leaders must take into account various elements to ensure they choose the right technology partner. Here are several considerations to keep in mind:
- Assessment of Needs: It's critical to assess your company's specific requirements. Different industries require tailored solutions to maximize NLP effectiveness.
- Evaluation of Technologies: Investigate the core technologies employed by the provider and their proficiency in those areas. A strong technological foundation may indicate future adaptability and performance.
- Long-term Viability: Companies must evaluate the long-term viability of the NLP provider. Check for ongoing support, updates, and developments to guarantee your partner will evolve alongside your needs.
- Feedback and Reviews: Gathering insights from existing customers can provide valuable perspectives on reliability, performance, and service quality.
By taking these factors into account, businesses, especially small to medium-sized enterprises, can make informed choices that align with their strategic goals and foster innovation.