Understanding Data Lake SaaS: Key Insights and Benefits
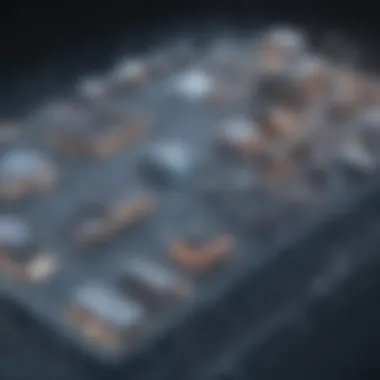
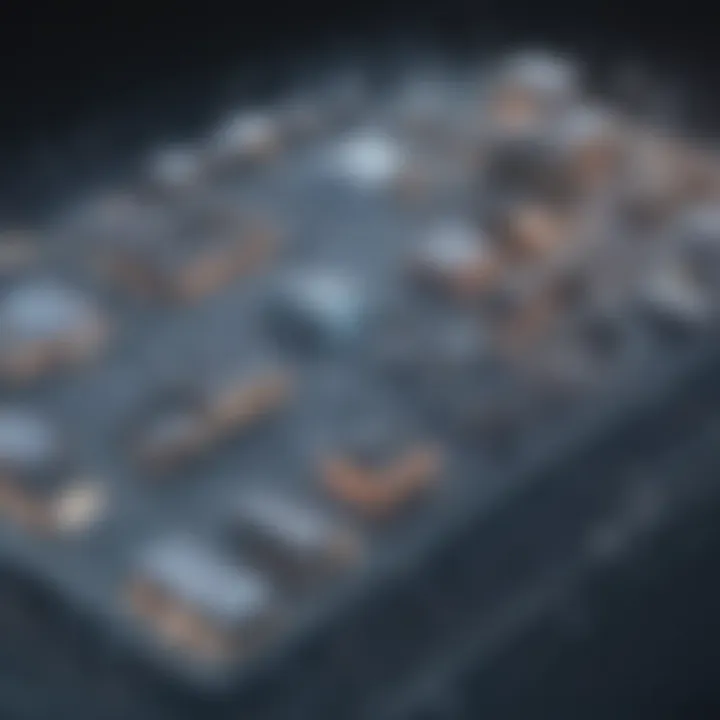
Intro
Data Lake Software as a Service (SaaS) is transforming how organizations manage and analyze their data. With the surge in digital data, companies across various sectors are recognizing the need for effective solutions to store, manage, and utilize vast amounts of information. In the current climate, data-driven decision-making is increasingly vital. Thus, understanding Data Lake SaaS can offer valuable insights for businesses eager to harness their data's potential.
The architecture of Data Lake SaaS provides a flexible and scalable approach. It allows organizations to store structured and unstructured data in its native format. As a result, companies can efficiently query and analyze data without the limitations imposed by traditional data warehousing.
Software Overview
Purpose of the Software
The primary purpose of Data Lake SaaS is to facilitate the storage, processing, and analysis of large volumes of data from diverse sources. This software model supports various functions, including data ingestion, transformation, and exploration. It is especially beneficial for organizations that wish to leverage data analytics for enhanced decision-making.
Data Lake SaaS platforms aim to break down data silos and create a unified data repository. This integration enables businesses to gain a comprehensive view of their operations, customers, and market trends. As organizations evolve, the need for a centralized data hub becomes even more important.
Key Features
Data Lake SaaS solutions come with several key features, including:
- Scalability: These platforms can easily scale to accommodate growing data needs without significant infrastructure changes.
- Flexibility: Users can store various data types seamlessly, allowing for more comprehensive analysis.
- Real-Time Processing: Many Data Lake solutions support real-time data ingestion and analysis, enabling timely insights.
- Cost Efficiency: By leveraging cloud infrastructure, organizations can reduce upfront costs and pay for only the resources consumed.
"Data Lake SaaS democratizes access to data, making it simpler for businesses to analyze not just what they know, but also what they don't yet understand."
Comparison with Competitors
Feature-by-Feature Analysis
When evaluating Data Lake SaaS options, it's crucial to compare their features against competitors. Each solution may offer distinct advantages. Key comparisons may include:
- Data Ingestion Speed: Some solutions excel at quickly incorporating vast amounts of data from various channels.
- Security Measures: The extent of security protocols in place can influence trust and compliance for sensitive data.
- User Experience: The design and ease of use of the software can affect adoption rates among team members.
Pricing Comparison
Pricing models for Data Lake SaaS vary widely across providers. Organizations should assess:
- Subscription Costs: Monthly or annual fees,
- Pricing Based on Usage: Costs may be tied to volume of data stored or processed.
- Hidden Fees: It is also important to evaluate potential costs related to scaling or data egress.
Defining Data Lakes and SaaS
Defining Data Lakes and SaaS is fundamental to understanding how modern businesses manage and utilize their data. As organizations evolve in the digital landscape, the way they store, process, and analyze data becomes increasingly important. This section outlines the essential concepts of Data Lakes and Software as a Service to establish a common framework for their integration and functionality.
Understanding Data Lakes
Data Lakes serve as vast repositories for storing a variety of data types. Unlike traditional databases, Data Lakes can accommodate structured, semi-structured, and unstructured data without needing extensive pre-processing. This flexibility allows businesses to gather large amounts of data without immediate constraints, making it easier to analyze trends and patterns over time. The primary advantage of a Data Lake is its ability to manage data from multiple sources and formats, facilitating a comprehensive view of information across an organization.
Moreover, the architecture of a Data Lake supports scalability. Businesses can continuously add more data without worrying about exceeding capacity or performance degradation. This aspect is particularly beneficial for small to medium-sized businesses that often face fluctuating data storage needs as they grow. In essence, Data Lakes enable organizations to harness their data effectively, promoting informed decision-making.
What is Software as a Service?
Software as a Service, commonly known as SaaS, refers to a cloud-based service where applications are hosted and managed by a third-party provider. This delivery model allows businesses to access software applications over the internet without the need for local installation or maintenance. As such, users can benefit from automatic updates and enhanced security without ongoing management.
The SaaS model is advantageous for many organizations, particularly for small and medium-sized businesses, because it reduces the upfront costs associated with software deployment. Instead of investing heavily in infrastructure and licenses, businesses can utilize a pay-as-you-go subscription model that aligns with their operational needs. This flexibility permits faster deployment, scalability, and easier integration with other cloud services.
The Intersection of Data Lakes and SaaS
Understanding the intersection of Data Lakes and SaaS is vital for businesses looking to leverage data in the cloud. Integrating Data Lakes within a SaaS framework allows for easier access and management of vast datasets. Businesses can store their data in a comprehensive, scalable Data Lake while employing SaaS applications to analyze that data effectively.
This combination enhances decision-making processes, as users can extract meaningful insights from their data without significant delays. SaaS providers often offer analytical tools that can securely connect to Data Lakes, thereby streamlining the process of data retrieval and processing.
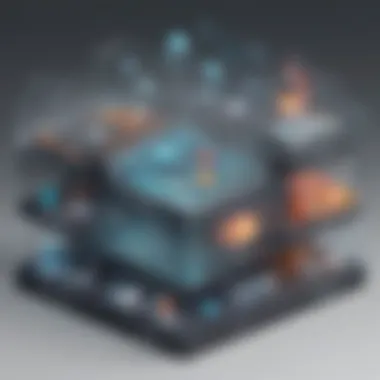
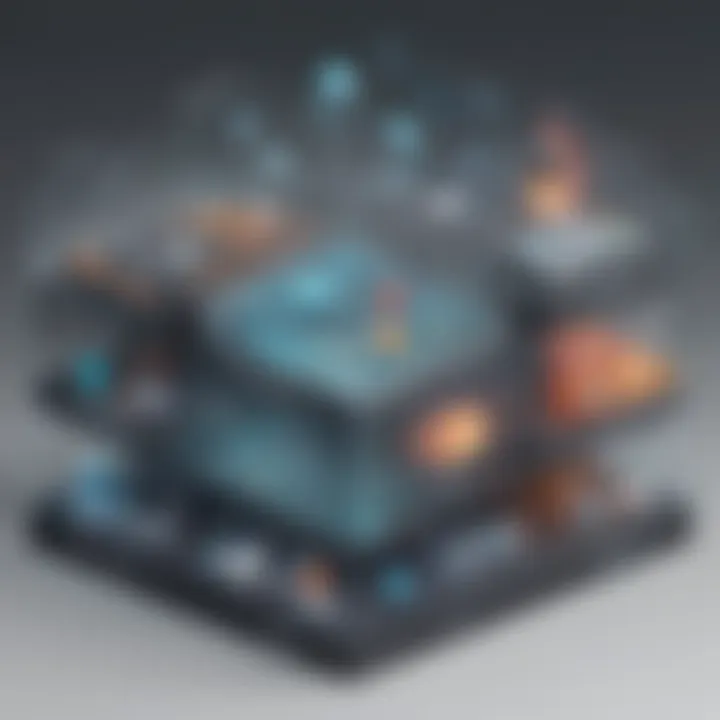
A notable benefit of this integration is the democratization of data access. Employees at various levels can interact with data stored in a Data Lake through user-friendly SaaS tools, improving collaboration across departments. Consequently, businesses can tap into broader insights and foster a culture of data-driven decision-making.
"By leveraging Data Lake SaaS solutions, organizations can harness a wealth of information quickly, adapting to market changes and operational demands more efficiently."
Core Components of Data Lake SaaS
The core components of Data Lake SaaS are fundamental for understanding how these systems function and deliver value to organizations. Each component plays a critical role in managing, processing, and securing vast amounts of data. The focus on these components helps businesses realize the full potential of their data lakes while addressing specific operational needs and concerns.
Data Ingestion Methods
Data ingestion encompasses the processes and techniques used to collect and import data from various sources into a data lake. Effective ingestion methods are essential for ensuring data is current, relevant, and actionable. There are two main types of data ingestion: batch ingestion, where data is collected and transferred at scheduled intervals, and real-time ingestion, which allows for immediate data processing.
Batch ingestion tends to work well for large datasets that do not require immediate action, while real-time ingestion supports scenarios needing instantaneous insights, such as online transaction processing. The choice of ingestion method depends on business needs, data velocity, and the type of data being processed. Additionally, well-implemented ingestion methods facilitate data consistency and reliability across the lake, which is key for effective analysis.
Storage Architectures
Storage architecture defines how data is organized, managed, and accessed within a data lake. A robust storage architecture supports both structured and unstructured data seamlessly. Storing data in a raw format allows organizations to leverage advanced analytics tools more efficiently. Traditionally, storage solutions have been hierarchical, but with the advent of cloud technology, scalable and flexible options are now predominant.
Common architectures include object storage, file systems, and relational databases. Cloud providers like Amazon Web Services, Microsoft Azure, and Google Cloud offer scalable storage solutions that grow with a company's data demands. Cost-effective and flexible storage architecture empowers businesses to manage data efficiently without incurring unnecessary expenses. The architecture chosen will impact performance, retrieval speed, and long-term profitability.
Data Processing Frameworks
A data lake's capability hinges significantly on the processing frameworks utilized to analyze the data stored within it. These frameworks allow organizations to perform complex data transformations and gain insights that can drive decision-making. Frameworks like Apache Spark, Apache Flink, and Apache Beam are popular due to their capabilities for large-scale data processing quickly.
Processing can be divided into batch processing and stream processing. Batch processing analyzes data in segments while stream processing provides near-real-time insights. The choice between these methods greatly influences responsiveness and the ability to act swiftly on insights gleaned from data. Moreover, having a seamless integration with machine learning and business intelligence tools enhances the overall analytical capabilities of the data lake.
Data Governance and Security
Data governance is a critical aspect of managing a data lake, as it ensures data integrity, compliance, and security. Organizations must establish policies to manage data usage, quality, and accessibility while ensuring adherence to regulations such as GDPR or HIPAA. An effective governance strategy involves creating access control policies, data lineage tracking, and cataloging data for easy identification and retrieval.
Security measures play a vital role in protecting sensitive data from unauthorized access and breaches. This includes data encryption, user authentication, and regular security assessments. Ensuring robust governance and security frameworks is paramount in building stakeholder trust and meeting legal obligations. In the long run, it aids in nurturing a culture of data-centric decision-making while safeguarding business reputation.
Benefits of Data Lake SaaS Solutions
Data Lake SaaS solutions present a dynamic approach to data management and analytics for businesses. Their relevance stems from their ability to address key challenges faced by organizations today. With data volumes increasing rapidly, finding scalable and efficient methods to handle this data is crucial. Data Lake SaaS solutions offer numerous benefits that support improved decision-making and operational efficiency. Below, we will explore these advantages in detail.
Scalability and Flexibility
One of the primary benefits of Data Lake SaaS solutions is scalability. Businesses can start with a small amount of data and expand as their needs grow. This aspect is vital for small to medium-sized enterprises that often work with limited budgets. They can invest in additional storage and processing power when the need arises, minimizing waste and optimizing resources.
Flexibility also accompanies scalability. Companies can adjust their data environments in real-time to accommodate changing data types and volumes. They can easily ingest various data formats, such as structured, semi-structured, and unstructured data. This adaptability means organizations can experiment with different data sources without major operational upheavals.
Cost-Effectiveness
Cost is a prominent consideration for businesses adopting new technology. Data Lake SaaS solutions typically operate on a subscription model, allowing businesses to pay for the services they use. This model is more predictable and manageable compared to traditional infrastructure investments, which might require significant upfront capital.
Moreover, Data Lake SaaS can lead to reduced operational costs. It typically requires less maintenance and fewer IT resources than on-premises systems. The service provider handles upgrades, security, and maintenance, allowing businesses to focus on using their data rather than managing the underlying infrastructure.
Faster Data Retrieval
Time is an essential asset in today’s competitive landscape. Data Lake SaaS enables faster data retrieval by utilizing optimized storage solutions and indexing strategies, significantly improving query performance. By processing data directly at the storage level, businesses experience quicker insights and more timely responses to market conditions.
The architecture of Data Lake SaaS also supports concurrent access to data. This is particularly beneficial for teams requiring quick responses or those working on collaborative projects. Enhanced data retrieval speeds can lead to a direct impact on decision-making processes and ultimately influence the organization's agility.
Enhanced Collaboration Capabilities
Collaboration across departments is essential for a holistic approach to data analysis. Data Lake SaaS solutions promote enhanced collaboration by providing a unified platform where teams can share data and insights effortlessly. With centralized access, different stakeholders can access the same data sets to make informed decisions.
Additionally, these platforms often include features that facilitate sharing and collaboration, such as dashboards and reporting tools. Teams can align their efforts more readily, improving efficiency and fostering a culture of data-driven decision-making.
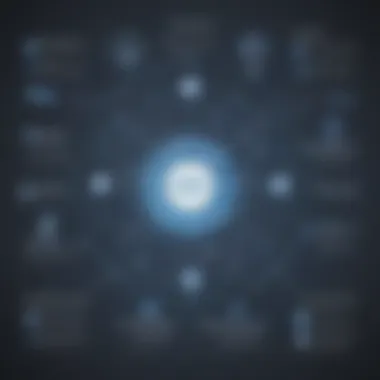
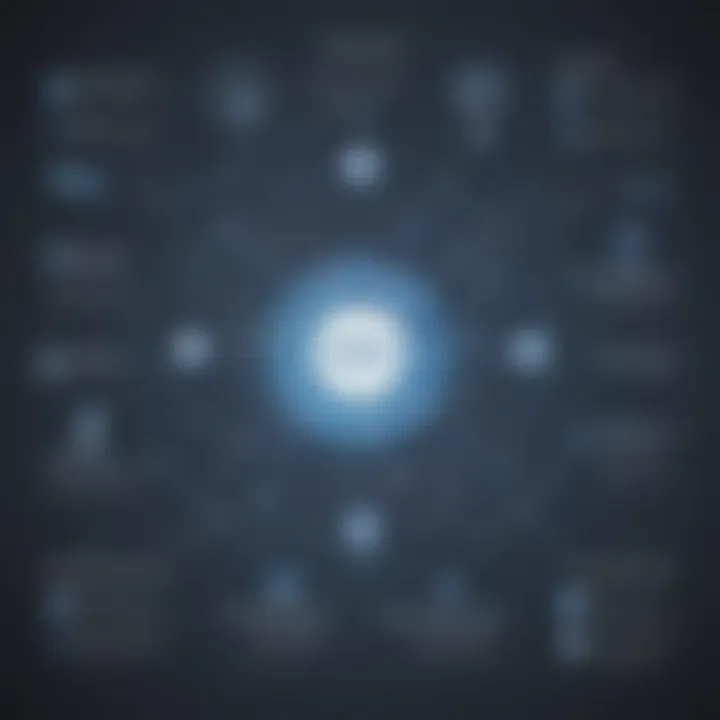
"Data Lake SaaS offers a framework where teams can thrive by utilizing shared resources effectively."
Challenges in Implementing Data Lake SaaS
Implementing Data Lake Software as a Service (SaaS) involves various complexities. Understanding these challenges is crucial for businesses seeking to derive the full benefits of data lakes while ensuring a smooth transition from traditional data management solutions. The urgency to harness data effectively must be balanced with a realistic understanding of possible obstacles that may arise in implementation.
Data Quality Management
Data quality management serves as a foundational element for successful data lake implementation. In a data lake, data is stored in its raw format. This flexibility is an advantage. However, it can also lead to inconsistencies. Poor data quality may compromise analytics outcomes, driving poor decision-making. Organizations must establish clear guidelines and processes for data entry, transformation, and storage. Data validation mechanisms should be in place to constantly monitor and ensure the integrity and accuracy of data. Regular audits and data cleanup initiatives can help maintain high data quality standards over time.
Integration with Existing Systems
Another challenge lies in integrating the data lake with existing systems. Many enterprises rely on a mix of legacy systems and modern applications. The complexity comes from ensuring seamless communication between these various systems and the new data lake. Organizations need to invest time to assess their current architecture and workflows. Choosing the right data integration tools is vital. It facilitates the movement of data between the lake and various applications without significant disruption. Moreover, effective integration can enhance the overall functionality of the data lake.
Compliance and Regulatory Considerations
Compliance with regulatory frameworks is also an essential challenge. Businesses must adhere to rules that govern data usage and storage, such as the GDPR or HIPAA. Failing to comply can result in serious repercussions, including hefty fines and damage to reputation. Organizations must have a thorough understanding of applicable regulations. Establishing robust governance policies is critical to comply with these frameworks. Regular training and updates on compliance issues can help in mitigating risks associated with these legal obligations.
User Training and Adoption
Lastly, user training is a significant challenge in implementing Data Lake SaaS. The success of any new solution heavily relies on user adoption. If employees find the system complex or cumbersome, it may not be used effectively. Organizations need to invest in comprehensive training programs tailored to different user roles. These programs should not only cover technical functionalities but also emphasize the importance of data-driven decision-making. Continuous support and feedback mechanisms can further encourage adoption and reduce resistance.
"A well-implemented data lake can be a game-changer, but understanding and addressing associated challenges is key to unlocking its potential."
In summary, while Data Lake SaaS solutions offer numerous benefits, acknowledging and addressing these implementation challenges is critical for success. From ensuring data quality to navigating compliance issues, it is essential for businesses to approach the implementation process thoughtfully.
Use Cases for Data Lake SaaS in Industry
Understanding the practical applications of Data Lake SaaS is essential for organizations aiming to harness data effectively. Each industry has unique challenges and opportunities, and Data Lake SaaS offers tailored solutions to meet these needs. The flexibility, scalability, and integration capabilities of Data Lake solutions make them increasingly relevant in today’s data-centric world. This section explores a few prominent industries leveraging Data Lake SaaS and the specific advantages that arise from their adoption.
Healthcare Sector Applications
The healthcare sector faces a vast amount of data daily. Patient records, treatment plans, research data, and medical imaging all contribute to a data deluge. Data Lake SaaS solutions provide healthcare facilities with the ability to consolidate and analyze this information seamlessly. With the help of these solutions, healthcare professionals can unlock insights that drive better patient outcomes, optimize resource use, and enhance overall operational efficiency.
Data Lake technology aids in predictive analytics, enabling hospitals to anticipate patient inflow and react accordingly. Moreover, it allows for the integration of data from disparate sources, such as electronic health records and wearable devices. This comprehensive view fosters data-driven decision-making, which is vital in enhancing patient care.
Financial Services and Banking
In the financial services sector, data integrity and speed are of paramount importance. Data Lake SaaS helps banks and financial institutions gather enormous data sets from transactions, credit reports, and market analytics. This capability enables organizations to develop better risk assessment models, fraud detection systems, and targeted marketing strategies.
For instance, banks can analyze historical transaction data against current spending patterns to flag unusual activities. This proactive approach to security not only protects assets but also enhances customer trust. Additionally, the ability to visualize and analyze financial data translates into improved regulatory compliance and smarter investment strategies.
Retail and E-Commerce Insights
Retail and e-commerce businesses thrive on their ability to understand consumer behavior and trends. Data Lake SaaS provides retailers with a powerful tool to collect data from various channels like online sales, in-store purchases, and social media interactions. This insight allows them to tailor marketing campaigns based on actual consumer behavior, leading to increased sales and customer satisfaction.
With the capability to run advanced analytics, retailers can also optimize inventory management. By predicting trends and customer demands, businesses can manage stock levels efficiently, reducing both excess inventory and stock-outs. This operational efficiency is essential in maintaining competitiveness in a fast-paced market.
Manufacturing Optimization
Manufacturing industries increasingly depend on data to enhance operational efficiency and product quality. Data Lake SaaS allows manufacturers to collect and analyze data from equipment sensors, production lines, and supply chain processes. This data-driven approach identifies inefficiencies and areas for improvement within the manufacturing process.
By utilizing machine learning algorithms on the data stored in the Data Lake, manufacturers can anticipate maintenance needs and avoid costly downtime. Additionally, real-time analytics enable immediate adjustments in production schedules based on supply chain disruptions or changes in demand.
"Data-driven insights in manufacturing not only increase efficiency but also improve product quality, leading to higher customer satisfaction."
Selecting the Right Data Lake SaaS Provider
Choosing an appropriate Data Lake SaaS provider is crucial for businesses aiming to harness the full potential of their data. The right choice impacts data management efficiency, operational agility, and ultimately, business outcomes. Therefore, it is essential for decision-makers to consider several factors when selecting a provider that aligns with their goals and requirements.
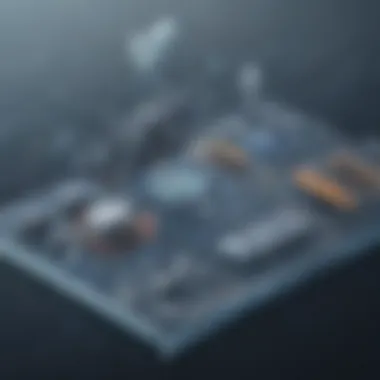
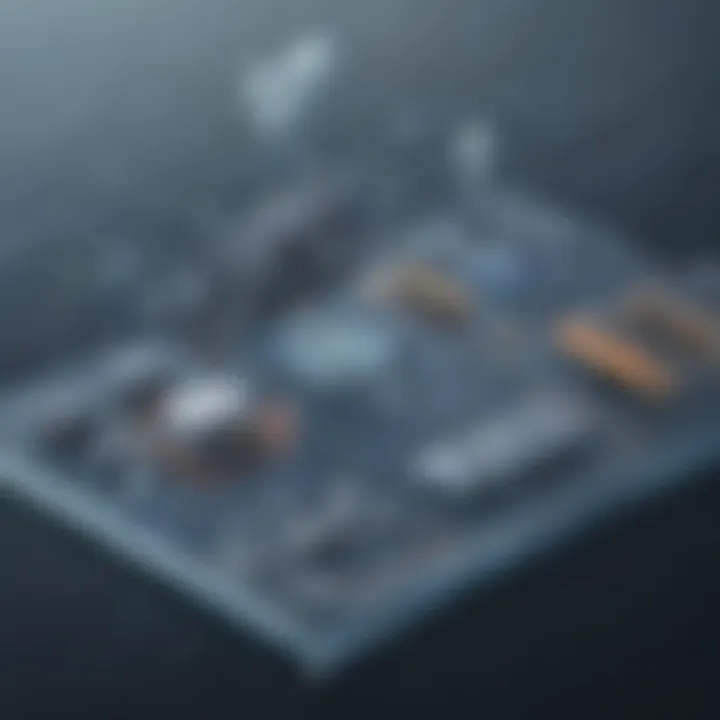
Key Evaluation Criteria
Several criteria can guide the evaluation of a Data Lake SaaS provider:
- Scalability: As businesses grow, their data needs will evolve. Ensure that the provider can accommodate increasing data volumes without performance degradation.
- Integration capabilities: Assess how well the provider’s solution can integrate with existing systems and tools. Seamless connectivity is vital for optimizing data flow across platforms.
- Data security: Security measures should be robust to protect sensitive information. Verify the provider's compliance with relevant regulations and standards.
- Performance: Evaluate the speed of data processing and retrieval. A solution that guarantees high performance can significantly enhance decision-making efficiency.
- Cost structure: Understand the pricing model. This includes not only initial costs but also potential long-term expenses as usage scales.
Comparing Top Providers
An effective approach in selecting a Data Lake SaaS provider involves comparing leading options available in the market. This helps identify which solutions offer the best fit for specific business needs. Key providers to consider include:
- Amazon Web Services (AWS) Lake Formation: Known for its comprehensive features and integration within the AWS ecosystem.
- Google Cloud Storage: Offers strong integration with Google’s analytics tools and reliable performance.
- Microsoft Azure Data Lake: Provides excellent scalability and strong data security features, ideal for enterprises already using Microsoft products.
Conduct thorough research on each solution’s strengths and weaknesses based on your organization’s unique requirements. Industry reviews and case studies can be useful resources in this comparison.
Customizability and Support Options
Customization capabilities are pivotal when selecting a Data Lake SaaS provider. Businesses have unique data structures and processes. A flexible platform allows for tailored configurations to suit specific needs. Furthermore, consider the support options provided by the vendor. Good customer support can resolve issues quickly, preventing potential disruptions to business operations. Check for:
- Availability of technical support: Ideally, look for 24/7 support to address urgent issues.
- Documentation and resources: Ensure ample resources are available for user training and troubleshooting. Strong documentation assists users in maximizing the platform's functionalities.
Selecting the right Data Lake SaaS provider is a strategic decision that requires careful consideration of various factors. With the right choice, businesses can effectively manage their data, leading to improved insights and informed decision-making.
Future Trends in Data Lake SaaS
The landscape of Data Lake SaaS is shifting rapidly, influenced by innovations and evolving business needs. Understanding these future trends is not just beneficial but crucial for small to medium-sized businesses, entrepreneurs, and IT professionals who aim to leverage data effectively. As companies implement or upgrade their data solutions, it becomes clear that foresight in technology developments can guide strategies for successful outcomes.
Emerging Technologies Impact
Emerging technologies are transforming the way organizations interact with data. Machine learning and artificial intelligence are at the forefront of this transformation. They enable better data analysis, driving more informed decision-making. By employing predictive analytics, companies can anticipate trends and customer needs rather than merely reacting.
Furthermore, advancements in cloud computing are simplifying infrastructure management. This enhances scalability and operational efficiency. For instance, tools like Amazon Web Services and Google Cloud Platform provide robust support for maintaining data lakes, facilitating cost-effective scaling as data needs grow. The integration of these technologies will likely lead to increased adoption of Data Lake SaaS solutions.
The Shift Towards Decentralized Architectures
As organizations look for greater flexibility, the shift to decentralized architectures is gaining momentum. Traditional centralized approaches often lead to bottlenecks. By adopting a decentralized model, data access becomes more agile and responsive to various business units. This can enhance collaboration and innovation.
In decentralized systems, data can be managed closer to where it is generated. This setup reduces latency and improves the real-time processing capabilities that many organizations strive for. Companies that implement decentralized data lakes will likely experience improved user satisfaction and better alignment of data use with business objectives.
Increased Importance of Data Privacy
The importance of data privacy will only continue to grow as regulatory frameworks evolve. Organizations will need to navigate laws such as the General Data Protection Regulation (GDPR) and the California Consumer Privacy Act (CCPA). Compliance requirements can become complex, especially with ample data flowing through a data lake.
Establishing robust data governance frameworks is essential for maintaining privacy and security. Data Lake SaaS solutions are increasingly incorporating features that provide automated compliance tracking and audit trails. Businesses must prioritize privacy management strategies that ensure data is handled ethically and in accordance with regulations. This not only protects businesses from legal repercussions but also builds trust with customers.
"The future of Data Lake SaaS is intertwined with the emergence of innovative technologies, and the importance placed on data privacy cannot be understated."
The End
In this article, we explored the intricate landscape of Data Lake Software as a Service. Understanding Data Lake SaaS is essential for small to medium-sized businesses, entrepreneurs, and IT professionals who aim to leverage vast amounts of data effectively. In an age where making informed decisions is crucial, harnessing the power of Data Lake solutions can greatly enhance operational efficiency and strategic planning.
Summarizing Key Insights
The importance of Data Lake SaaS lies in its ability to consolidate different data forms all in one platform. Throughout the discussion, we highlighted several core components:
- Data Ingestion Methods: These methods pave the way for seamless data entry into the lake.
- Storage Architectures: Choosing the right architecture ensures that data is stored effectively, facilitating easy access.
- Data Governance and Security: Ensuring that data governance is managed well is critical to maintain compliance and security standards.
- Cost-Effectiveness: Often, these solutions present a better financial model than traditional data management systems.
The insights gathered through this exploration indicate that integrating Data Lake SaaS can deliver significant competitive advantages in various sectors, including healthcare, finance, and retail.
Final Thoughts on Data Lake SaaS
In closing, the rise of Data Lake SaaS represents a transformative opportunity for businesses ready to innovate. As industries continue to adapt and evolve in response to data proliferation, adopting a robust data management strategy is no longer optional; it is necessary.
The journey into the world of Data Lake SaaS can appear complex, yet the rewards justify the efforts required to understand and implement it. For businesses aiming to remain relevant and competitive, incorporating Data Lake solutions is a step toward achieving effective, data-driven decision-making.
"The future of data management lies within the efficient structure and accessibility that Data Lake SaaS offers."
As organizations prioritize digital transformation, embracing Data Lake SaaS will be critical to extracting valuable insights from their growing data reserves.