Understanding Labelbox Segmentation: A Comprehensive Guide
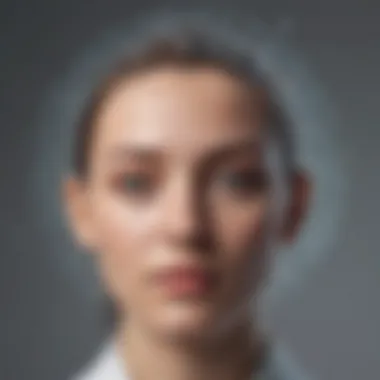
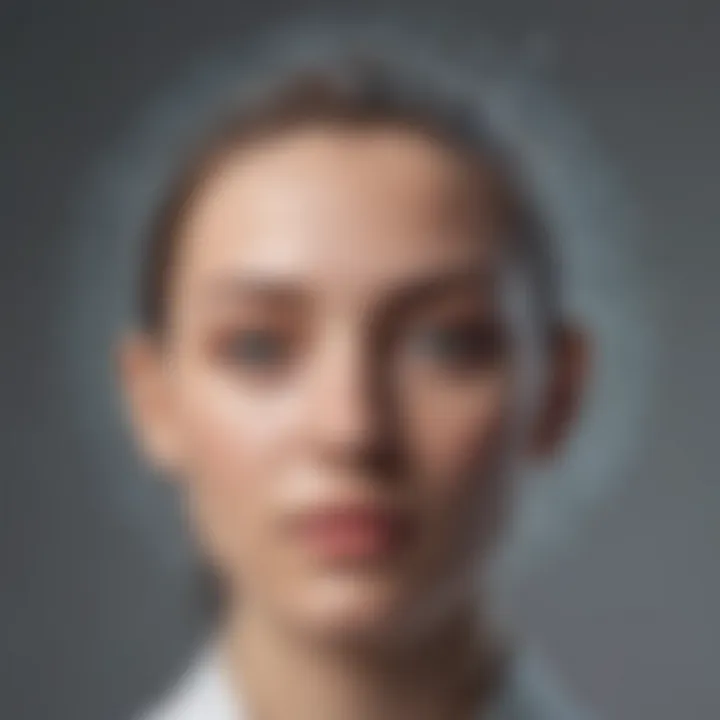
Intro
In today's data-driven world, machine learning and artificial intelligence play crucial roles. Effective data annotation holds the key to successful model training. Within this context, Labelbox emerges as a noteworthy tool, enabling efficient data management and annotation through advanced segmentation. This analysis aims to break down the segmentation features of Labelbox, demonstrating how they can be harnessed to optimize data annotation processes for both small and medium-sized businesses.
Understanding the significance of segmentation is essential to appreciate its impact on the entire data preparation workflow. As businesses strive to enhance their machine learning models, the methodical organization of training data becomes paramount. Labelbox supports users in accomplishing this through intuitive segmentation functionalities that streamline their tasks and improve labeling accuracy.
Software Overview
Purpose of the Software
Labelbox is designed to facilitate the data annotation process effectively. Its interface allows users to create, manage, and annotate datasets with precision. Segmenting data is essential in distinguishing between different categories or attributes, which helps machine learning models to generalize better from the training data. Thus, Labelbox provides a platform where users can manage their segmentation efforts seamlessly.
Key Features
Labelbox offers several key features that make it stand out in the data annotation landscape:
- Custom Segmentation Tools: Users have the ability to create tailored segmentation tools, which allows for specific categorization of data, fitting unique project needs.
- Collaboration Capabilities: The platform encourages collaboration among team members. Multiple users can annotate and segment data concurrently, enhancing efficiency.
- Quality Assurance Mechanisms: Labelbox implements quality control features that ensure the integrity and accuracy of the annotations provided by its users.
- Integration Support: It supports integration with various machine learning frameworks, ensuring a smooth workflow from data annotation to model training.
These features, among others, provide businesses with the necessary tools to enhance their data annotation strategies.
Comparison with Competitors
Labelbox faces competition in the data annotation space from other platforms like Amazon SageMaker, Supervisely, and VGG Image Annotator. Understanding how it compares is vital for businesses considering its adoption.
Feature-by-Feature Analysis
Here’s a snapshot of how Labelbox compares with its competitors on key features:
- User Interface: Labelbox offers a user-friendly experience that makes navigation easier for new users compared to Amazon SageMaker, which can be more complex.
- Collaboration: Labelbox excels in collaboration options, allowing teams to work simultaneously. This feature may be less pronounced in competitors.
- Segmentation Options: Custom segmentation tools in Labelbox are more robust than many alternatives, enabling tailored approaches for various projects.
Pricing Comparison
When it comes to pricing, Labelbox provides flexible plans depending on business needs. Some competitors might offer lower initial costs but can become expensive with additional features. Labelbox users appreciate transparency in its pricing model, making budgeting more straightforward. For organizations managing budgets wisely, this clarity is a considerable benefit.
"Labelbox provides clarity and focus on segmentation, which is crucial for effective data management and machine learning training purposes."
Preface to Labelbox Segmentation
Understanding Labelbox segmentation is crucial for businesses looking to optimize their data annotation processes. This section will delve into the importance of Labelbox as a tool for segmentation in machine learning, highlighting the unique benefits it brings to data professionals and organizations.
Labelbox serves as a powerful platform that facilitates effective data labeling, which is foundational for training accurate machine learning models. By providing an intuitive interface and extensive customization options, it enables users to annotate images, videos, and other data types seamlessly. This ease of use can lead to significant time savings and enhanced productivity. Additionally, the collaborative features of Labelbox allow team members to share insights and feedback, fostering a more agile workflow.
What is Labelbox?
Labelbox is a comprehensive data annotation platform designed to assist in creating high-quality labeled datasets for machine learning projects. It caters to various industries, including healthcare, automotive, and retail, thereby emphasizing its versatility. The platform enables users to perform a range of annotation tasks—from image segmentation to video tracking—with a user-friendly interface that minimizes the learning curve for new users.
Moreover, Labelbox employs advanced technologies such as AI-assisted annotation, which speeds up the labeling process while ensuring accuracy. This combination of features positions Labelbox as a vital resource for businesses aiming to enhance their machine learning capabilities.
Overview of Segmentation
Segmentation refers to the process of dividing an image into meaningful regions. In the context of Labelbox, segmentation plays a critical role in identifying and labeling distinct elements within a dataset. This capability is especially important in applications such as object detection, where accurate delineation of objects can significantly affect the performance of machine learning models.
Labelbox's segmentation tools allow users to define regions with precision, enabling detailed annotations that cater to the specific needs of their projects. Users can choose from various segmentation methods, including polygon, box, and freehand tools, a variety that further enriches the annotation process.
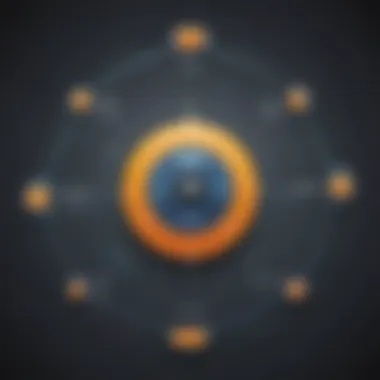
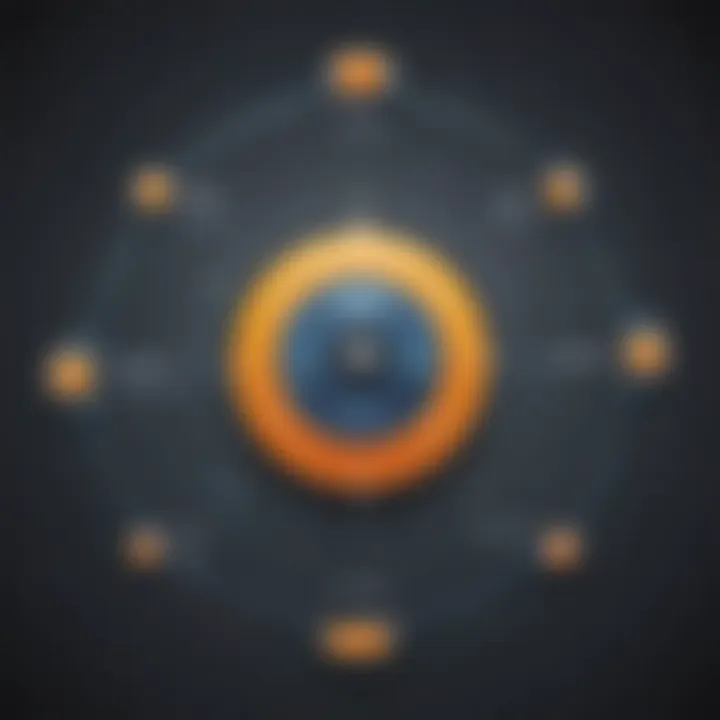
In summary, understanding Labelbox segmentation equips businesses with the knowledge necessary to utilize this tool effectively. By leveraging its features, they can improve data quality, enhance machine learning models, and ultimately achieve better outcomes.
The Importance of Data Annotation
Data annotation is a crucial aspect in the development of machine learning models and artificial intelligence applications. It enables machines to interpret and learn from vast amounts of data, making sense of unstructured information. With the rise of machine learning technologies, the role of quality data annotation has gained prominence. This section explores the significance of data annotation, particularly focusing on its role in machine learning and the enhancement of AI models.
Role in Machine Learning
Machine learning primarily relies on data to train models. The process of training involves using labeled data to teach algorithms how to make decisions. Data annotation provides the backbone for these labeled datasets. Without precise annotation, models cannot learn effectively, leading to inaccurate predictions and faulty decision-making processes.
- Quality Control: It ensures that the data used for training is reliable. Poorly labeled data leads to errors during training, which impacts model performance.
- Facilitating Learning: Annotation helps machines understand the context of the input data. For example, in computer vision, labeling an image allows the model to discern between a cat and a dog.
- Adaptability: As enterprises grow, data annotation allows scalability. Instead of starting from scratch, models can adapt to new data and environments with pre-annotated datasets.
This highlights how pivotal data annotation is in forming the foundation upon which machine learning operates. It is not merely a supplementary step; it is instrumental in shaping the learning journey of algorithms.
Enhancing AI Models
The objectives of machine learning can only be achieved through accurately annotated data. Enhanced AI models perform better due to the quality of training data, which directly correlates to data annotation. Enhanced training leads to better performance in several domains.
- Increased Accuracy: High-quality annotations reduce misinformation, leading to more accurate predictions. This accuracy is fundamental in fields like healthcare or finance.
- Rich Feature Extraction: Annotated data helps models to capture subtle patterns. In natural language processing, for instance, proper labeling enables the model to understand sentiment and context.
- Reduction in Bias: Careful annotation can help identify and mitigate biases. This is essential to create fair AI systems that do not perpetuate historical inequalities.
"The success of AI models is highly contingent upon the quality of their training data. Without proper data annotation, even the most advanced algorithms may fail to deliver expected results."
Key Features of Labelbox Segmentation
Labelbox segmentation offers an array of features designed to enhance the data annotation process, making it a powerful tool for businesses working in artificial intelligence and machine learning. Understanding these features is crucial as they determine how effectively a team can annotate data. Labelbox aims to streamline workflows while providing robust functionalities that meet the unique needs of various industries. In this section, we will explore the key attributes that make Labelbox segmentation stand out, focusing on usability, customization, collaboration, and data management capabilities.
User-Friendly Interface
One of the main advantages of Labelbox is its user-friendly interface. Designed with the intention of minimizing the learning curve, this platform allows users to navigate effortlessly through its functionalities. The intuitive layout ensures that even those with limited technical expertise can engage with the software efficiently. Functions are clearly labeled, and tools are logically grouped, which facilitates a seamless annotation experience. This ease of use is particularly beneficial for small to medium-sized businesses looking to onboard new team members quickly without extensive training.
Customizable Annotation Tools
Customization is another strong feature of Labelbox segmentation. Users can tailor the annotation tools to fit their specific project needs, thereby enhancing both accuracy and efficiency. The platform supports diverse annotation types, from bounding boxes to polygons. This adaptability allows teams to work on a variety of data forms, including images and videos. Furthermore, users can create their own templates for recurring tasks, saving precious time in data processing. This flexibility is crucial for projects with unique requirements, enabling businesses to maintain high standards of quality in their data labeling efforts.
Collaboration Capabilities
Collaboration is essential when working on data annotation projects that involve multiple stakeholders. Labelbox facilitates this through its built-in collaboration features. Team members can easily share projects, provide feedback, and communicate directly within the platform. This functionality not only enhances the workflow but also helps in maintaining consistency in annotations. Additionally, project managers can track progress in real-time, allowing them to manage resources more effectively. The ability to work collectively in a centralized space makes Labelbox particularly appealing to teams that favor a cooperative approach.
Data Management Features
Effective data management is at the heart of successful annotation processes, and Labelbox provides solid tools for this purpose. The platform allows users to organize their data efficiently, enabling quick access and retrieval. Users can categorize datasets and apply filters to ease the navigation of large volumes of information. Additionally, Labelbox supports integration with various data storage solutions, streamlining the data flow from collection to annotation. Security features also play a vital role, ensuring sensitive data is protected while being annotated. This focus on data management contributes significantly to optimizing the workflow and improving the overall quality of the machine learning models being developed.
"Effective segmentation can significantly accelerate the development of AI models, and this is where Labelbox excels."
Applications of Segmentation in Business
Segmentation is a crucial aspect of data annotation, particularly in business applications. It allows organizations to tailor their machine learning models to specific needs. The process segments data into meaningful portions, increasing the model's accuracy and performance. Incorporating segmentation into business strategies can lead to enhanced decision-making and more targeted solutions. This section will delve into various use cases across different industries and explore how segmentation can seamlessly fit into existing workflows.
Use Cases in Various Industries
Segmentation finds applications in numerous sectors. Here are some notable examples:
- Healthcare: In medical imaging, segmentation helps identify and isolate specific structures, such as organs or tumors. This aids in diagnosis and treatment planning.
- Retail: Retailers use segmentation to analyze customer behavior. By understanding purchasing patterns, they can tailor marketing strategies and improve customer experience.
- Automotive: In autonomous driving, segmentation is vital for object detection. It helps identify pedestrians, vehicles, and road signs, ensuring safer navigation.
- Agriculture: Farmers can utilize segmentation to analyze crop health through aerial imaging. This allows for targeted interventions and resource optimization.
Each of these applications highlights how segmentation can drive efficiency and outcomes in diverse industries, leveraging data to create specific solutions.
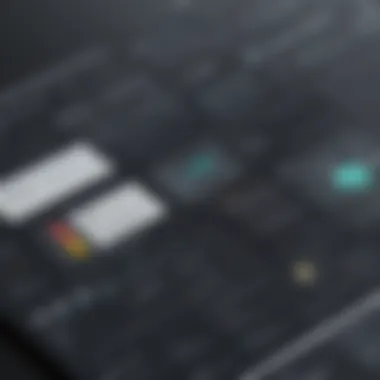
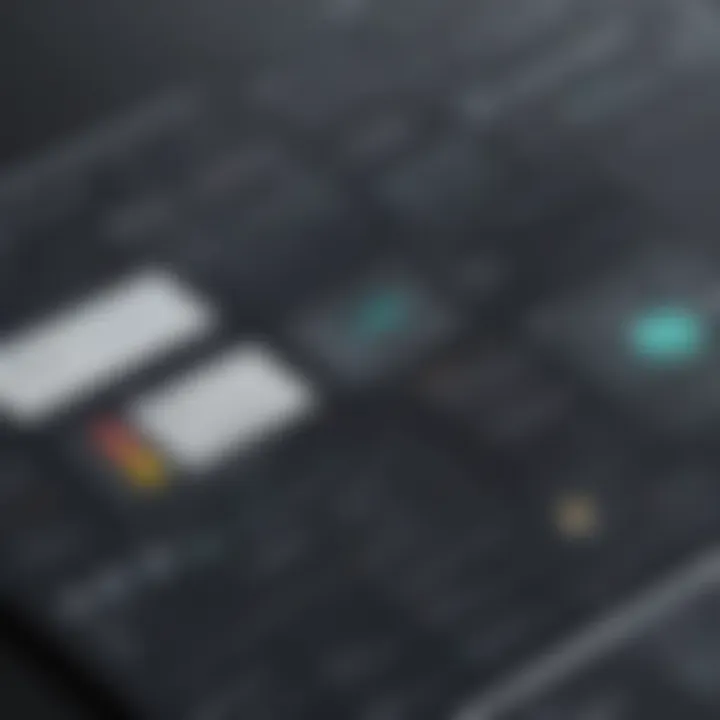
Integration with Existing Workflows
Integrating segmentation into existing workflows is essential for maximizing its benefits. Here are some strategies to consider:
- Identify Key Processes: Recognize areas where segmentation can add value, such as data collection and analysis workflows.
- Leverage Existing Tools: Use systems already in place, like CRM or ERP platforms, to incorporate segmentation seamlessly.
- Train Stakeholders: Educate team members on the advantages of segmentation. This ensures buy-in and enhances user adoption.
- Iterate and Refine: Regularly review the segmentation processes. Adapt and improve based on feedback and results.
By being aware of how segmentation aligns with current practices, organizations can enhance productivity and generate actionable insights.
"Successful integration of segmentation into workflows leads to a more agile and responsive operation."
Best Practices for Implementing Segmentation
When implementing segmentation within Labelbox, understanding best practices can dramatically enhance the results achieved through data annotation. These practices guide users in optimizing their approaches to segmenting data, ultimately leading to superior machine learning outcomes.
Defining Clear Objectives
Defining clear objectives is the first step in any segmentation project. It sets the direction for the entire annotation process. Businesses should begin by asking key questions: What specific problem are we addressing? What insights do we desire from segmenting this data? By having specific goals, teams can make focused decisions that align with the intended outcome. Without defined objectives, projects may lack guidance, resulting in disorganized annotation efforts that do not meet business needs.
Some essential considerations include:
- Understanding the end-user requirements for segmentation.
- Identifying the type of data that will be segmented.
- Defining how the segmented data will be used in machine learning models.
Choosing the Right Data Types
Choosing appropriate data types for segmentation is crucial for achieving effectiveness in the annotation process. Different datasets require unique strategies for segmentation. For example, image data might demand pixel-level annotation, while text data might require entity recognition.
Factors to consider here include:
- The nature of the dataset (text, image, video, etc.).
- The segmentation techniques that are suitable for each data type.
- Compatibility of the data with the existing workflows and tools.
By selecting the right data types, businesses can ensure that their segmentation efforts are relevant and yield useful results.
Ensuring Annotation Quality
Quality of annotation directly affects the performance of machine learning models. Therefore, ensuring high annotation quality is non-negotiable. This involves implementing quality control measures throughout the annotation process.
Some methods to enhance annotation quality include:
- Training annotators on specific standards and guidelines.
- Regularly reviewing annotations for consistency.
- Utilizing advanced tools within Labelbox for validation processes.
A good approach is to encourage feedback and make adjustments based on the insights gained from quality assessment. By prioritizing annotation quality, organizations can significantly improve the accuracy and reliability of their data, which is paramount for successful machine learning applications.
"Properly implemented segmentation processes lead to higher-quality data, which is the foundation for reliable machine learning models."
Common Challenges and Solutions
In the realm of data annotation, challenges are omnipresent. Addressing these challenges is crucial for the effective utilization of Labelbox segmentation. Recognizing these obstacles aids in maintaining high standards in data quality and efficiency. This section will highlight major challenges users face along with potential solutions, fostering a deeper understanding of how to navigate the complexities of data segmentation.
Identifying Inconsistencies
Inconsistencies in data labeling can lead to significant issues in model training. These inconsistencies manifest in various forms, including mislabeling, ambiguous definitions, and varying formats by different annotators. The importance of identifying these inconsistencies cannot be overstated. A well-annotated dataset is essential for the accuracy and reliability of machine learning models.
Here are several techniques to detect and manage inconsistencies:
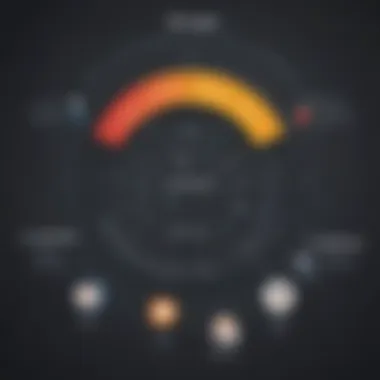
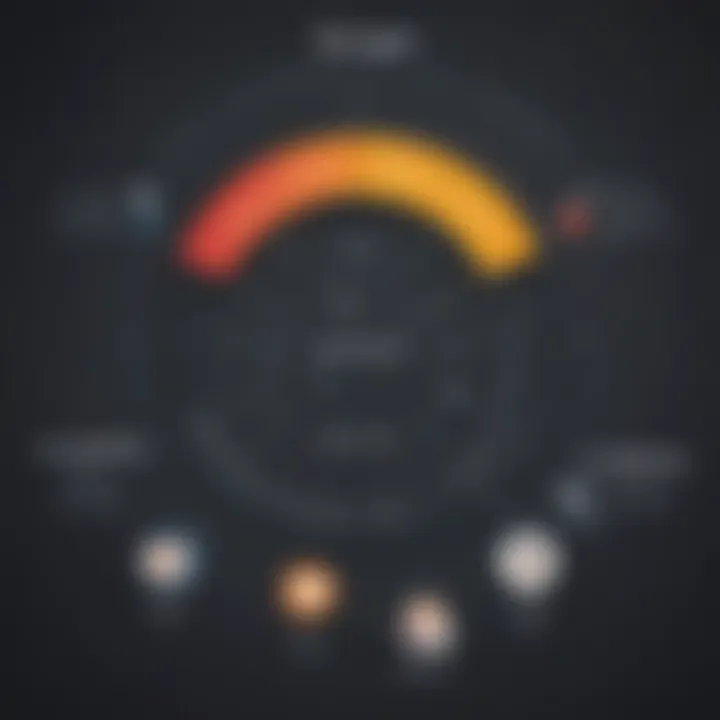
- Regular Audits: Conduct frequent reviews of annotated data to catch inconsistencies early. Spot checks can save time and resources in the long run.
- Automated Tools: Leverage AI-powered tools within Labelbox to flag potential errors. These tools can analyze patterns in data and provide alerts on probable inconsistencies.
- Standard Annotation Guidelines: Establish and communicate clear guidelines for annotators to follow. This minimizes variation in labeling.
"Detecting inconsistencies early can save your projects time, effort, and financial resources."
By placing a strong emphasis on identifying inconsistencies within annotated data, businesses can significantly enhance their data quality.
Addressing Scalability Issues
As projects grow, scalability becomes a crucial concern. Many businesses may initially manage data annotation well, but they often find themselves overwhelmed as volume increases. Labelbox segmentation provides tools to help mitigate these scalability challenges. However, implementing effective solutions is vital to ensuring smooth operations as demand rises.
Consider the following strategies for addressing scalability:
- Optimizing Workflow: Streamline processes to reduce bottlenecks. An organized workflow can help manage high volume efficiently.
- Collaborative Environment: Foster a collaborative platform where multiple team members can work simultaneously. Labelbox’s collaboration features can facilitate this.
- Infrastructure Scaling: Ensure that your technology infrastructure can support increasing data loads. Cloud solutions often offer flexibility in this area.
By proactively tackling scalability issues, organizations can expand their data annotation processes without sacrificing quality or efficiency. Taking these steps helps businesses harness the full potential of Labelbox segmentation.
Future Trends in Data Annotation and Segmentation
The field of data annotation and segmentation is rapidly evolving. Understanding these trends is essential for businesses looking to maintain a competitive edge in the marketplace. As AI technologies develop, so does the landscape in which they operate. Organizations are increasingly realizing the need to adapt their annotation strategies to incorporate new methodologies that enhance efficiency and accuracy. The significance of this evolution cannot be overstated, as it directly influences the effectiveness of machine learning models and, ultimately, business outcomes.
Advancements in AI and Automation
In recent years, substantial advancements in AI have transformed data annotation processes. Automated tools are now able to handle complex tasks that previously required manual intervention. Tools powered by machine learning and deep learning algorithms provide a level of efficiency that can dramatically reduce the time and cost associated with annotation work. This progress allows for scaling annotation projects that can handle large datasets.
- Model Training: With automation, the systems can learn from previous annotations to improve future outputs. This adaptive learning enhances precision.
- Quality Assurance: Automated systems can conduct initial reviews, flag inconsistencies, and suggest corrections, thus streamlining the quality control process.
- Increased Throughput: Annotators can focus on more critical aspects of the work, leaving repetitive tasks to machines, resulting in higher overall productivity.
These improvements are essential for businesses that rely on accurate data for AI model training. Without them, enterprises risk falling behind competitors who embrace newer technologies.
The Role of Human Oversight
While automation is vital, it is equally important to acknowledge the role of human oversight in the annotation process. Automated systems, although efficient, can sometimes misinterpret nuanced data or fail to understand contextual elements. Thus, human input remains necessary to ensure that the annotations are not only accurate but also relevant and useful for the intended applications.
- Critical Decision Making: Humans provide insights into data that machines might overlook, particularly in complex scenarios where contextual understanding is crucial.
- Ethical Considerations: Human oversight helps address ethical issues in data use, ensuring that models are not inadvertently biased and that they comply with regulations.
- Enhancing Model Performance: Combining human insight with automated tools can create a more robust annotation framework, enhancing the quality of data fed into machine learning systems.
"Human oversight in automated processes is not an option but a necessary blend to achieve optimal outcomes in data annotation."
Finale
The conclusion serves as a pivotal component of this article. Here, we encapsulate the core principles and insights pertaining to Labelbox segmentation. Understanding segmentation not only elucidates its functional value but also highlights the multitude of ways it can optimize data annotation processes. One must recognize that effective segmentation is integral to achieving superior data quality, which is foundational in any machine learning initiative.
When summarizing the value of Labelbox segmentation, we consider its specific contributions:
- Increased Efficiency: Labelbox's tools streamline the annotation process, enabling faster project completions.
- Enhanced Accuracy: The provided features help in minimizing human error, a crucial factor in machine learning success.
- Scalability: Companies can handle larger data sets with ease, presenting opportunities for growth and innovation.
These benefits coalesce to create a framework that empowers small to medium-sized businesses and professionals in they journey toward integrating complex machine learning systems. The importance of grasping these points cannot be understated as they influence decision-making and strategic planning within organizations.
"Labelbox segmentation paves the way for precision in machine learning implementations, helping businesses harness their data potential."
Summarizing the Value of Labelbox Segmentation
Labelbox’s segmentation capabilities are designed to meet the rising demands for precision in data annotation. By effectively utilizing these features, businesses ensure that their data is not only annotated quickly but also with a high standard of quality. This dual focus fosters stronger AI models that can perform better in practical scenarios.
Moreover, as companies adopt these annotation techniques, they gain insights into their own data ecosystems. Understanding the intricacies of their data leads to better-informed decisions and strategies. In summary, leveraging Labelbox's segmentation enhances the overall data lifecycle, providing a robust foundation for machine learning endeavors. Therefore, embracing these functionalities is not merely a choice but a strategic necessity for any aspiring entity in this competitive digital age.
Final Thoughts for Businesses
As businesses evaluate their options for enhancing data annotation, the significance of tools like Labelbox becomes clear. The ability to segment data effectively will redefine how organizations approach their machine learning projects. Firms should prioritize exploring these capabilities to stay competitive.
Investing time in learning and implementing Labelbox segmentation can yield dividends in various dimensions, including efficiency, accuracy, and adaptability. In an era where data informs decisions at all levels, it is imperative to ensure the quality of that data through effective annotation practices. Therefore, businesses that take these factors into account are not just improving their operations; they are positioning themselves for sustained success in an increasingly data-driven world.
By adopting the insights and practices outlined in this article, companies can navigate the complex landscape of machine learning with greater confidence and depth. This intentional approach will ultimately contribute to a more informed, intelligent interaction with data, leading to better outcomes in their projects.